Abstract
Students employing problem-based learning (PBL) face complex problems admitting multiple solutions. Knowledge maps are an effective tool to support students in PBL to represent, analyze, and guide their knowledge structure. Previous studies have examined the use of knowledge mapping techniques in both individual and collaborative learning process. These studies explored how knowledge mapping influenced students’ learning outcomes and their knowledge understanding, but they did not document how the map structure changed when shifting from individual to collaborative mapping process. In this work-in-process study, we collected maps from students first as individuals and then as they collaboratively worked in groups to solve a case. We examined two questions: (Q1) how does the structure of a map change when shifting from individuals to groups, and (Q2) how does the size of a group mediate these changes. We used 12 network science metrics capturing the breadth (e.g., number of edges) and complexity (e.g., number of cycles) of problems and compared these metrics in individuals versus groups (Q1) and across group sizes (Q2). Based on 44 individual maps arranged in 10 groups of four or five students, we found that (1) five metrics significantly differ (p < 0.05 in a one-way ANOVA) between individual and group maps; and (2) only the number of edges and paths are affected by the size of a group (based on Tukey's HSD test). Our first result confirms that learner-learner interactions can enhance performance. The second result shows that group sizes of four or five students have no implications on the results, hence instructors who may currently be hesitating between these two options may choose either size. Future studies may reveal whether differences exist between pairs, medium group sizes, or larger groups.







Similar content being viewed by others
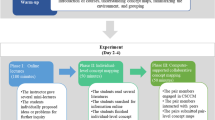
References
Ballard, J., & Mooring, S. R. (2021). Cleaning our world through green chemistry: Introducing high school students to the principles of green chemistry using a case-based learning module. Journal of Chemical Education, 98(4), 1290–1295. https://doi.org/10.1021/acs.jchemed.9b00312
Burns, H. (2022). Imagining imagination: Towards cognitive and metacognitive models. Pedagogy, Culture and Society. https://doi.org/10.1080/14681366.2022.2059547
Cacciamani, S., Perrucci, V., & Fujita, N. (2021). Promoting students’ collective cognitive responsibility through concurrent, embedded and transformative assessment in blended higher education courses. Technology, Knowledge and Learning, 26, 1169–1194. https://doi.org/10.1007/s10758-021-09535-0
Cline, B. E., Brewster, C. C., & Fell, R. D. (2010). A rule-based system for automatically evaluating student concept maps. Expert Systems with Applications, 37(3), 2282–2291. https://doi.org/10.1016/j.eswa.2009.07.044
Dayu, D. P. K., Pratiwi, C. P., & Hakim, P. R. (2022). Problem-based learning model to increase students’ critical thinking. Jurnal Basicedu, 6(3), 4671–4680. https://doi.org/10.31004/BASICEDU.V6I3.2842
Eseryel, D., Ifenthaler, D., & Ge, X. (2013). Validation study of a method for assessing complex ill-structured problem solving by using causal representations. Educational Technology Research and Development, 61(3), 443–463. https://doi.org/10.1007/S11423-013-9297-2
Farrokhnia, M., Pijeira-Díaz, H. J., Noroozi, O., & Hatami, J. (2019). Computer-supported collaborative concept mapping: The effects of different instructional designs on conceptual understanding and knowledge co-construction. Computers and Education, 142, 103640. https://doi.org/10.1016/j.compedu.2019.103640
Frerichs, L., Young, T. L., Dave, G., Stith, D., Corbie-Smith, G., & Hassmiller Lich, K. (2018). Mind maps and network analysis to evaluate conceptualization of complex issues: A case example evaluating systems science workshops for childhood obesity prevention. Evaluation and Program Planning, 68, 135–147. https://doi.org/10.1016/J.EVALPROGPLAN.2018.03.003
Freund, A. J., & Giabbanelli, P. J. (2021). Are we modeling the evidence or our own biases? A comparison of conceptual models created from reports. Annual Modeling and Simulation Conference (ANNSIM), 2021, 1–12.
Giabbanelli, P. J., & Tawfik, A. A. (2019). Overcoming the PBL assessment challenge: Design and development of the incremental thesaurus for assessing causal maps (ITACM). Technology, Knowledge and Learning, 24(2), 161–168.
Giabbanelli, P. J., & Tawfik, A. A. (2020). Reducing the gap between the conceptual models of students and experts using graph-based adaptive instructional systems. In HCI International 2020–Late Breaking Papers: Cognition, Learning and Games: 22nd HCI International Conference, HCII 2020, Copenhagen, Denmark, July 19–24, 2020, Proceedings 22 (pp. 538–556). Springer International Publishing.
Giabbanelli, P. J., & Tawfik, A. A. (2021). How perspectives of a system change based on exposure to positive or negative evidence. Systems, 9(2), 23.
Giabbanelli, P. J., Tawfik, A. A., & Wang, B. (2022). Designing the next generation of map assessment systems: Open questions and opportunities to automatically assess a student’s knowledge as a map. Journal of Research on Technology in Education. https://doi.org/10.1080/15391523.2022.2119449
Gupta, V. K., Giabbanelli, P. J., & Tawfik, A. A. (2018). An online environment to compare students’ and expert solutions to ill-structured problems. In Learning and Collaboration Technologies. Learning and Teaching: 5th International Conference, LCT 2018, Held as Part of HCI International 2018, Las Vegas, NV, USA, July 15–20, 2018, Proceedings, Part II 5 (pp. 286–307). Springer International Publishing.
Gutiérrez-Braojos, C., Rodríguez-Domínguez, C., Daniela, L., et al. (2023). An analytical dashboard of collaborative activities for the knowledge building. Technology, Knowledge and Learning. https://doi.org/10.1007/s10758-023-09644-y
Hmelo-Silver, C. E. (2004). Problem-based learning: What and how do students learn? Educational Psychology Review, 16(3), 235–266. https://doi.org/10.1023/B:EDPR.0000034022.16470.F3
Hmelo-Silver, C. E., & Eberbach, C. (2012). Learning theories and problem-based learning. In S. Bridges, C. McGrath, & T. L. Whitehill (Eds.), Problem-Based learning in clinical education: The next generation (pp. 3–17). Springer. https://doi.org/10.1007/978-94-007-2515-7_1
Huang, K., Ge, X., & Eseryel, D. (2017). Metaconceptually-enhanced simulation-based inquiry: Effects on eighth grade students’ conceptual change and science epistemic beliefs. Educational Technology Research and Development, 65(1), 75–100. https://doi.org/10.1007/S11423-016-9462-5/TABLES/8
Ifenthaler, D. (2014). AKOVIA: Automated Knowledge Visualization and Assessment. Technology, Knowledge and Learning, 19, 241–248. https://doi.org/10.1007/s10758-014-9224-6
Ifenthaler, D., Masduki, I., & Seel, N. M. (2011). The mystery of cognitive structure and how we can detect it: Tracking the development of cognitive structures over time. Instructional Science, 39(1), 41–61.
Jeong, A., Li, H., & Pan, A. J. (2017). A sequential analysis of responses in online debates to postings of students exhibiting high versus low grammar and spelling errors. Educational Technology Research and Development, 65(5), 1175–1194. https://doi.org/10.1007/S11423-016-9501-2/FIGURES/7
Jeong, H., & Hmelo-Silver, C. E. (2016). Seven affordances of computer-supported collaborative learning: How to support collaborative learning? How can technologies help? Educational Psychologist, 51(2), 247–265. https://doi.org/10.1080/00461520.2016.1158654
Kao, G. Y. M., Lin, S. S. J., & Sun, C. T. (2008). Breaking concept boundaries to enhance creative potential: Using integrated concept maps for conceptual self-awareness. Computers and Education, 51(4), 1718–1728. https://doi.org/10.1016/J.COMPEDU.2008.05.003
Kim, K., Clarianay, R. B., & Kim, Y. (2019). Automatic representation of knowledge structure: Enhancing learning through knowledge structure reflection in an online course. Educational Technology Research and Development, 67(1), 105–122.
Kim, M. K., Gaul, C. J., Kim, S. M., et al. (2020). Advance in detecting key concepts as an expert model: Using student mental model analyzer for research and teaching (SMART). Technology, Knowledge and Learning, 25, 953–976. https://doi.org/10.1007/s10758-019-09418-5
Kooloos, J. G. M., Klaassen, T., Vereijken, M., Van Kuppeveld, S., Bolhuis, S., & Vorstenbosch, M. (2011). Collaborative group work: Effects of group size and assignment structure on learning gain, student satisfaction and perceived participation. Medical Teacher, 33(12), 983–988. https://doi.org/10.3109/0142159X.2011.588733
Krabbe, H. (2014). Digital concept mapping for formative assessment. In D. Ifenthaler & R. Hanewald (Eds.), Digital knowledge maps in education (pp. 275–297). Springer.
Ligabo, M., Carvalho Silva, F., da Carvalho, A. C. S. A., Rodrigues, D., & Rodrigues, R. C. L. B. (2023). Practical way to apply fourth-generation assessment tools integrated into creating meaningful learning experiences in biology at high school. Evaluation and Program Planning, 96, 102155. https://doi.org/10.1016/j.evalprogplan.2022.102155
Ma, C., & Zhou, W. (2022). Effects of unfolding case-based learning on academic achievement, critical thinking, and self-confidence in undergraduate nursing students learning health assessment skills. Nurse Education in Practice, 60, 103321. https://doi.org/10.1016/j.nepr.2022.103321
McKinney, W. (2011). pandas: A foundational Python library for data analysis and statistics. Python for High Performance and Scientific Computing, 14(9), 1–9.
Nesbit, J. C., & Adesope, O. O. (2016). Learning with concept and knowledge maps: A meta-analysis. Review of Educational Research, 76(3), 413–448. https://doi.org/10.3102/00346543076003413
Oliván-Blázquez, B., Aguilar-Latorre, A., Gascón-Santos, S., Gómez-Poyato, M. J., Valero-Errazu, D., Magallón-Botaya, R., Heah, R., & Porroche-Escudero, A. (2022). Comparing the use of flipped classroom in combination with problem-based learning or with case-based learning for improving academic performance and satisfaction. Active Learning in Higher Education. https://doi.org/10.1177/14697874221081550
Ouyang, F., Wu, M., Zhang, L., Xu, W., Zheng, L., & Cukurova, M. (2023). Making strides towards AI-supported regulation of learning in collaborative knowledge construction. Computers in Human Behavior. https://doi.org/10.1016/j.chb.2023.107650
Ouyang, F., & Xu, W. (2022). The effects of three instructor participatory roles on a small group’s collaborative concept mapping. Journal of Educational Computing Research, 60(4), 930–959. https://doi.org/10.1177/0735633121105728
Pfister, H. R., & Oehl, M. (2009). The impact of goal focus, task type and group size on synchronous net-based collaborative learning discourses. Journal of Computer Assisted Learning, 25(2), 161–176. https://doi.org/10.1111/J.1365-2729.2008.00287.X
Reed, S. K. (2016). The structure of ill-structured (and well-structured) problems revisited. Educational Psychology Review, 28(4), 691–716. https://doi.org/10.1007/S10648-015-9343-1
Rong, H., & Choi, I. (2019). Integrating failure in case-based learning: A conceptual framework for failure classification and its instructional implications. Educational Technology Research and Development, 67(3), 617–637. https://doi.org/10.1016/j.learninstruc.2012.05.003
Rosba, E., Zubaidah, S., Mahanal, S., & Bin Jamaluddin, A. (2023). Student creativity through online learning using digital mind map-assisted group investigation. AIP Conference Proceedings, 2569, 020014. https://doi.org/10.1063/5.0112375
Savery, J. R. (2006). Overview of problem-based learning: Definitions and distinctions. Interdisciplinary Journal of Problem-Based Learning, 1(1), 9–20. https://doi.org/10.7771/1541-5015.1002
Schau, C., Mattern, N., Zeilik, M., Teague, K. W., & Weber, R. J. (2016). Select-and-fill-in concept map scores as a measure of students’ connected understanding of science. Educational and Psychological Measurement, 61(1), 136–158. https://doi.org/10.1177/00131640121971112
Schroeder, N. L., Nesbit, J. C., Anguiano, C. J., & Adesope, O. O. (2018). Studying and constructing concept maps: A meta-analysis. Educational Psychology Review, 30(2), 431–455. https://doi.org/10.1007/s10648-017-9403-9
Shin, H. S., & Jeong, A. (2021). Modeling the relationship between students’ prior knowledge, causal reasoning processes, and quality of causal maps. Computers and Education, 163, 104113. https://doi.org/10.1016/J.COMPEDU.2020.104113
Si, J., Kong, H. H., & Lee, S. H. (2019). Developing clinical reasoning skills through argumentation with the concept map method in medical problem-based learning. Interdisciplinary Journal of Problem-Based Learning, 13(1), 5. https://doi.org/10.7771/1541-5015.1776
Sun, M., Wang, M., Wegerif, R., & Peng, J. (2022). How do students generate ideas together in scientific creativity tasks through computer-based mind mapping? Computers and Education, 176, 104359. https://doi.org/10.1016/J.COMPEDU.2021.104359
Tawfik, A. A., Giabbanelli, P. J., Hogan, M., Msilu, F., Gill, A., & York, C. S. (2017). Effects of success v failure cases on learner-learner interaction. Computers and Education, 118, 120–132. https://doi.org/10.1016/j.compedu.2017.11.013
Tawfik, A. A., Hung, W., & Giabbanelli, P. J. (2020a). Comparing how different inquiry-based approaches impact learning outcomes. Interdisciplinary Journal of Problem-Based Learning, 14(1), 1–17. https://doi.org/10.14434/IJPBL.V14I1.28624
Tawfik, A. A., Kim, K., & Kim, D. (2020b). Effects of case library recommendation system on problem solving and knowledge structure development. Educational Technology Research and Development, 68(3), 1329–1353. https://doi.org/10.1007/S11423-020-09737-W/TABLES/5
Tawfik, A. A., & Kolodner, J. L. (2016). Systematizing scaffolding for problem-based learning: A view from case-based reasoning. Interdisciplinary Journal of Problem-Based Learning, 10(1), 6. https://doi.org/10.7771/1541-5015.1608
Wang, B., & Giabbanelli, P. J. (2023). Identifying informative features to evaluate student knowledge as causal maps. International Journal of Artificial Intelligence in Education. https://doi.org/10.1007/s40593-023-00329-2
Wang, M., Wu, B., Kirschner, P. A., & Spector, J. M. (2018). Using cognitive mapping to foster deeper learning with complex problems in a computer-based environment. Computers in Human Behavior, 87, 450–458. https://doi.org/10.1016/j.chb.2018.01.024
Wilson, M., Howell, C., Martin-Morales, K., & Park, S. (2023). Concept mapping and reading comprehension. Journal of Political Science Education. https://doi.org/10.1080/15512169.2023.2164861
Yang, F., Lin, W., & Wang, Y. (2021). Flipped classroom combined with case-based learning is an effective teaching modality in nephrology clerkship. BMC Medical Education, 21(1), 1–7. https://doi.org/10.1186/s12909-021-02723-7
Yue, M., Zhang, M., Zhang, C., & Jin, C. (2017). The effectiveness of concept mapping on development of critical thinking in nursing education: A systematic review and meta-analysis. Nurse Education Today, 52, 87–94. https://doi.org/10.1016/J.NEDT.2017.02.018
Zabolotna, K., Malmberg, J., & Järvenoja, H. (2023). Examining the interplay of knowledge construction and group-level regulation in a computer-supported collaborative learning physics task. Computers in Human Behavior. https://doi.org/10.1016/j.chb.2022.107494
Fiume, A. F., Sciarrone, F., & Temperini, M. (2023). A new metric to help teachers unveil meaningful learning in concept maps. In International conference in methodologies and intelligent systems for technology enhanced learning (pp. 65–74). Springer. https://doi.org/10.1007/978-3-031-20617-7_9
Hagberg, A., Swart, P., & S Chult, D. (2008). Exploring network structure, dynamics, and function using NetworkX.
Jeong, A. (2010). Assessing change in learner’s causal understanding using sequential analysis and causal maps. In Learning in the disciplines: ICLS 2010 conference proceedings—9th international conference of the learning sciences (Vol. 1, pp. 73–80). https://doi.org/10.1007/978-1-4419-6530-1_11/TABLES/2
Lavin, E. A., Giabbanelli, P. J., Stefanik, A. T., Gray, S. A., & Arlinghaus, R. (2018). Should we simulate mental models to assess whether they agree? In Proceedings of the annual simulation symposium (pp. 1–12).
Saqr, M., Nouri, J., & Jormanainen, I. (2019). A learning analytics study of the effect of group size on social dynamics and performance in online collaborative learning. In Lecture notes in computer science (including subseries lecture notes in artificial intelligence and lecture notes in bioinformatics), 11722 LNCS (pp. 466–479). https://doi.org/10.1007/978-3-030-29736-7_35/TABLES/3
Shute, V. J., & Zapata-Rivera, D. (2008). Using an evidence-based approach to assess mental models. In Understanding models for learning and instruction (Vol. 25, pp. 23–41). https://doi.org/10.1007/978-0-387-76898-4_2
Strautmane, M. (2012). Concept map-based knowledge assessment tasks and their scoring criteria: An overview. In Proceedings of the 5th international conference on concept mapping (pp. 80–88).
Funding
The authors declare that no funds, grants, or other support were received during the preparation of this manuscript.
Author information
Authors and Affiliations
Contributions
BW: pre-processed, analyzed the collected data, and wrote the first draft of manuscript. AAT: wrote parts of the introduction, liaised between institutional teams, and reviewed this manuscript. CWK: collected the data from the University of Missouri Trulaske College of Business. PJG: designed this work-in-progress study, supervised BW work, and edited the first draft of the manuscript, and revised the manuscript.
Corresponding author
Ethics declarations
Conflict of interest
The authors have no relevant financial or non-financial interests to disclose.
Additional information
Publisher's Note
Springer Nature remains neutral with regard to jurisdictional claims in published maps and institutional affiliations.
Supplementary Information
Below is the link to the electronic supplementary material.
Rights and permissions
Springer Nature or its licensor (e.g. a society or other partner) holds exclusive rights to this article under a publishing agreement with the author(s) or other rightsholder(s); author self-archiving of the accepted manuscript version of this article is solely governed by the terms of such publishing agreement and applicable law.
About this article
Cite this article
Wang, B., Tawfik, A.A., Keene, C.W. et al. Transitioning from Individuals to Groups in Knowledge Map Construction. Tech Know Learn 29, 229–251 (2024). https://doi.org/10.1007/s10758-023-09651-z
Accepted:
Published:
Issue Date:
DOI: https://doi.org/10.1007/s10758-023-09651-z