Abstract
Beans usually have similar physical attributes; thus, it is difficult to distinguish them manually. Size, shape, and mass attributes of seeds help in breeding, selection, classification, separation, and machine design. This study was conducted to determine physical attributes of 20 bean genotypes with the use of image processing techniques. Color characteristics of the present genotypes were also determined. Then, four different machine learning algorithms (MLP, RF, SVR, and k-NN) were employed to predict seed mass. Among the present genotypes, Güzelöz and Özdemir genotypes had the highest size, shape, and color characteristics. Highly significant positive correlations were encountered between projected area-equivalent diameter (r = 1.00), between geometric mean diameter—surface area and volume (r = 1.00). On the other hand, highly significant negative correlations were seen between sphericity—elongation in vertical orientation (r = − 0.98). In hierarchical cluster analysis for physical attributes, Alberto–Aslan and Aras 98–Şahin genotypes were identified as the closest genotypes. According to PCA analysis, the first two principal components (PC1 and PC2) were able to explain 73% of total variation among the genotypes. While PC1 axis included projected area (vertical), equivalent diameter (vertical), and length, PC2 axis included L*, a*, b*, sphericity, roundness (vertical), and elongation (vertical). Among the present machine learning algorithms, RF yielded the best performances in mass estimation of bean seeds. It was concluded that machine learning techniques increased the efficiency of related machinery and helped to save time and labor.




Similar content being viewed by others
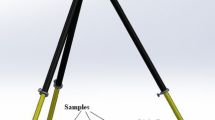
Data availability
The datasets generated during and/or analyzed during the current study are available from the corresponding author on reasonable request.
References
Çetin, M. (2007). Physical properties of barbunia bean (Phaseolus vulgaris L. cv.‘Barbunia’) seed. Journal of Food Engineering, 80(1), 353–358.
Çetin, N. (2022). Machine learning for varietal binary classification of soybean (Glycine max (L.) Merrill) seeds based on shape and size attributes. Food Analytical Methods. https://doi.org/10.1007/s12161-022-02286-3
Çetin, N., Karaman, K., Beyzi, E., Sağlam, C., & Demirel, B. (2021). comparative evaluation of some quality characteristics of sunfower oilseeds (Helianthus annuus L.) through machine learning classifers. Food Analytical Methods, 14, 1666–1681.
Çetin, N., Ozaktan, H., Uzun, S., Uzun, O., & Ciftci, C. Y. (2023). Machine learning based mass prediction and discrimination of chickpea (Cicer arietinum L.) cultivars. Euphytica, 219(1), 20.
Cetin, N., Yaman, M., Karaman, K., & Demir, B. (2020). Determination of some physicomechanical and biochemical parameters of hazelnut (Corylus avellana L.) cultivars. Turkish Journal of Agriculture and Forestry, 44(5), 439–450.
Chavoshgoli, E., Abdollahpour, S., Abdi, R., & Babaie, A. (2014). Aerodynamic and some physical properties of sunflower seeds as affected by moisture content. Agricultural Engineering International: CIGR Journal, 16(2), 136–142.
Colton, T. (1974). Statistics in medicine (p. p179). Little Brown and Co.
Demir, B., Eski, İ, Gürbüz, F., Kuş, Z. A., Sesli, Y., & Ercişli, S. (2020). Prediction of walnut mass based on physical attributes by Artificial Neural Network (ANN). Erwerbs-Obstbau, 62(1), 47–56.
Demir, B., Sayıncı, B., Çetin, N., Yaman, M., Çömlek, R., Aydın, Y., & Sütyemez, M. (2018). Elliptic Fourier based analysis and multivariate approaches for size and shape distinctions of walnut (Juglans regia L.) cultivars. Grasas y Aceites, 69(4), e271.
Fıratlıgil-Durmuş, E., Šárka, E., Bubník, Z., Schejbal, M., & Kadlec, P. (2010). Size properties of legume seeds of different varieties using image analysis. Journal of Food Engineering, 99(4), 445–451.
Flores, F., Nadal, S., Solis, I., Winkler, J., Sass, O., Stoddard, F. L., Link, W., Raffiot, B., Muel, F., & Rubiales, D. (2012). Faba bean adaptation to autumn sowing under European climates. Agronomy for Sustainable Development, 32(3), 727–734.
Food and Agriculture Organization of the United Nations (FAO) (2019). http://www.fao.org/faostat/en/#data/QC Accessed date, 06.01.2022.
George, C., McGruder, R., & Torgerson, K. (2007). Determination of optimal surface area to volume ratio for thin-layer drying of breadfruit (Artocarpus altilis). International Journal for Service Learning in Engineering, Humanitarian Engineering and Social Entrepreneurship, 2, 76–88.
Gonzalez, J. P. B., Ortiz, F. A. P., & Rodriguez, C. P. P. (2017). Mass and volume estimation of passion fruit using digital images. IEEE Latin America Transactions, 15(2), 275–282.
Guidoti, D. T., Gonela, A., Vidigal, M. C. G., Conrado, T. V., & Romani, I. (2018). Interrelationship between morphological, agronomic and molecular characteristics in the analysis of common bean genetic diversity. Acta Scientiarum. Agronomy. https://doi.org/10.4025/actasciagron.v40i1.33032
Gürbüz, F., Demir, B., Eski, I., Kuş, Z. A., Yilmaz, K. U., Ilikçioğlu, E., & Ercişli, S. (2018). Estimation of the weights of almond nuts based on physical properties through data mining. Notulae Botanicae Horti Agrobotanici Cluj-Napoca, 46(2), 579–584.
Güzel, D., & Sayar, S. (2012). Effect of cooking methods on selected physicochemical and nutritional properties of barlotto bean chickpea faba bean and white kidney bean. Journal of Food Science and Technology, 49(1), 89–95.
Hornberg, A. (2007). Handbook of machine vision. Wiley-VCH Verlag GmbH & Co KGaA.
Huang, M., Tang, J., Yang, B., & Zhu, Q. (2016). Classification of maize seeds of different years based on hyperspectral imaging and model updating. Computers and Electronics in Agriculture, 122, 139–145.
Jayant, M., Sahu, N. P., Deo, A. D., Garg, C. K., Yadav, R., & Gupta, S. (2021). Effective valorization of agro-waste of castor oil extraction industry as feedstock for sustainable fish production. Biofuels, Bioproducts and Biorefining, 15(4), 1126–1140.
Kara, M., Sayinci, B., Elkoca, E., Öztürk, İ, & Özmen, T. B. (2013). Seed size and shape analysis of registered common bean (Phaseolus vulgaris L.) cultivars in Turkey using digital photography. Journal of Agricultural Science, 19, 219–234.
Kibar, H., Öztürk, T., & Temizel, K. E. (2014). Effective engineering properties in the design of storage structures of postharvest dry bean grain. Acta Scientiarum. Agronomy, 36, 147–158.
Kliestik, T., Zvarikova, K., & Lăzăroiu, G. (2022). Data-driven machine learning and neural network algorithms in the retailing environment: Consumer engagement, experience, and purchase behaviors. Economics, Management and Financial Markets, 17(1), 57–69.
Kovacova, M., & Lăzăroiu, G. (2021). Sustainable organizational performance, cyber-physical production networks, and deep learning-assisted smart process planning in Industry 4.0-based manufacturing systems. Economics, Management and Financial Markets, 16(3), 41–54.
Kubálek, J., Čámská, D., & Strouhal, J. (2017). Personal bankruptcies from macroeconomic perspective. International Journal of Entrepreneurial Knowledge, 5(2), 78–88.
Maroušek, J., & Gavurová, B. (2022). Recovering phosphorous from biogas fermentation residues indicates promising economic results. Chemosphere, 291, 133008.
Maroušek, J., Strunecký, O., & Maroušková, A. (2022). Insect rearing on biowaste represents a competitive advantage for fish farming. Reviews in Aquaculture, 2022, 1–12.
McClean, P. E., & Raatz, B. (2017). Common bean genomes: Mining new knowledge of a major societal crop. In M. Pérez de la Vega, M. Santalla, & F. Marsolais (Eds.), The Common Bean Genome. Compendium of Plant Genomes (pp. 129–145). Springer, Cham.
McGuire, R. G. (1992). Reporting of objective colour measurements. HortScience, 27(12), 1254–1255.
Nogueira, A. F., Moda-Cirino, V., Delfini, J., Brandão, L. A., Mian, S., Constantino, L. V., & Azeredo Gonçalves, L. S. (2021). Morpho-agronomic, biochemical and molecular analysis of genetic diversity in the Mesoamerican common bean panel. PLoS ONE, 16(4), e0249858.
Oral, E., Kendal, E., & Doğan, Y. (2018). Bazı durum buğday çeşitlerinin biplot ve ammi (ana etkiler ve çarpimsal interaksiyonlar) analizleri ile stabilitelerinin belirlenmesi. Bahri Dağdaş Bitkisel Araştırma Dergisi, 7(1), 1–13.
Ozaktan, H. (2021). Technological characteristics of chickpea (Cicer arietinum L.) cultivars grown under natural conditions. Turkish Journal of Field Crops, 26(2), 235–243.
Ozaktan, H., & Doymaz, A. (2022). Mineral composition and technological and morphological performance of beans as influenced by organic seaweed-extracted fertilizers applied in different growth stages. Journal of Food Composition and Analysis, 114, 104741.
Ozaktan, H., Uzun, S., Uzun, O., & Ciftci, C. Y. (2022). Change in chemical composition and morphological traits of chickpea (Cicer arietinum L.) genotypes grown under natural conditions. Gesunde Pflanzen. https://doi.org/10.1007/s10343-022-00741-z
Ozturk, I., Kara, M., Elkoca, E., & Ercisli, S. (2009b). Physico-chemical grain properties of new common bean cv.‘Elkoca-05.’ Scientific Research and Essays, 4(2), 88–93.
Ozturk, I., Kara, M., Ercisli, S., & Kantar, F. (2010). Physical and chemical grain properties of new registered common bean cv.‘Kantar-05.’ Sains Malaysiana, 39(5), 725–730.
Ozturk, I., Kara, M., Yildiz, C., & Ercisli, S. (2009a). Physico-mechanical seed properties of the common Turkish bean (Phaseolus vulgaris) cultivars ‘Hinis’ and ‘Ispir.’ New Zealand Journal of Crop and Horticultural Science, 37(1), 41–50.
Parker, J. R. (2001). Rank and response combination from confusion matrix data. Information Fusion, 2(2), 113–120.
Rad, M. R. N., Fanaei, H. R., & Rad, M. R. P. (2015). Application of Artificial Neural Networks to predict the final fruit weight and Random Forest to select important variables in native population of melon (Cucumis melo L.). Scientia Horticulturae, 181, 108–112.
Rad, M. R. N., Ghalandarzehi, A., & Koohpaygani, J. A. (2017). Predicting eggplant ındividual fruit weight using an Artificial Neural Network. International Journal of Vegetable Science, 23(4), 331–339.
Rahman, A., & Cho, B. K. (2016). Assessment of seed quality using non-destructive measurement techniques: A review. Seed Science Research, 26(4), 285–305.
Saglam, C., & Cetin, N. (2022). Prediction of pistachio (Pistacia vera L.) mass based on shape and size attributes by using machine learning algorithms. Food Analytical Methods, 15(3), 739–750.
Sastry, D. V. S. S. R., Upadhyaya, H. D., & Srinivas, T. R. (2019). Variation for seed physical and hydration properties of chickpea (Cicer arietinum L.) mini core collection and their relevance to conservation and utilization. Plant Genetic Resources: Characterization and Utilization, 17(4), 311–324.
Sayıncı, B., Kara, M., Ercişli, S., Duyar, Ö., & Ertürk, Y. (2015). Elliptic Fourier analysis for shape distinction of Turkish hazelnut cultivars. Erwerbs-Obstbau, 57, 1–11.
Şehirali, S. (1988). Yemeklik Dane Baklagiller. Ankara Üniversitesi Ziraat Fakültesi Yayınları, 1089, Ders Kitabı, 314. Ankara.
Soares, J. D. R., Pasqual, M., Lacerda, W. S., Silva, S. O., & Donato, S. L. R. (2013). Utilization of artificial neural networks in the prediction of the bunches’ weight in banana plants. Scientia Horticulturae, 155, 24–29.
Witten, I. H., & Frank, E. (2005). Data mining, practical machine learning tools and techniques (525) (2nd ed.). Elsevier.
Funding
This study was supported by Turkish Scientific Research Council (TUBITAK) with the project number of 119O226.
Author information
Authors and Affiliations
Contributions
HO contributed to investigation, resources, and writing—original draft; NÇ contributed to conceptualization, data curation, methodology, software, visualization, and writing—original draft; SU contributed to formal analysis and editing; OU contributed to resources and formal analysis; CYC contributed to supervision and editing.
Corresponding author
Ethics declarations
Conflict of interest
Authors declare that he has no conflict of interest.
Consent to participate
Informed consent is not applicable in this study.
Ethical approval
This study does not contain any studies with human participants or animals performed by any of the authors.
Additional information
Publisher's Note
Springer Nature remains neutral with regard to jurisdictional claims in published maps and institutional affiliations.
Rights and permissions
Springer Nature or its licensor (e.g. a society or other partner) holds exclusive rights to this article under a publishing agreement with the author(s) or other rightsholder(s); author self-archiving of the accepted manuscript version of this article is solely governed by the terms of such publishing agreement and applicable law.
About this article
Cite this article
Ozaktan, H., Çetin, N., Uzun, S. et al. Prediction of mass and discrimination of common bean by machine learning approaches. Environ Dev Sustain (2023). https://doi.org/10.1007/s10668-023-03383-x
Received:
Accepted:
Published:
DOI: https://doi.org/10.1007/s10668-023-03383-x