Abstract
To guarantee a dignified life in low-incoming countries where socio-environmental negative implications derived from the municipal solid waste (MSW) by its excessive generation, lack of governance, and financial resources, sustainable and integrated solutions are required. From this perspective, well-located transfer stations (TSs) are conceived as an alternative to improve MSW management quality in municipalities that generate little MSW and have long transportation distances. Consequently, this research aims to find optimum areas to locate TSs in the province of Azuay-Ecuador as a commonwealth proposal. It applies a multi-criteria decision analysis based on geographic information systems with the analytical hierarchical process technique: a heuristic and scalable method that simultaneously evaluates subjective and non-monetized inputs. The authors faced it from a holistic perspective in a three-level hierarchical structure; starting from categories: technical, environmental, economic, and social; then criteria: rivers, protected areas, populated areas, land use, educational centers, health centers, tourist sites, basic services, roads, gravity centers, geology, power grid, and slopes; and finally, sub-criteria defined by criteria´s measurable attributes. This research provides local decision-makers with middle-scale tools and a first diagnosis to focus later efforts in local studios over potential sites. The results showed that 79.12% of the study area was restrictive to place a TS. The remaining territory suitability index values between 13 and 89% were found, with possible TSs at Paute, Sigsig, and Nabón to serve nine from fifteen cantons.
Similar content being viewed by others
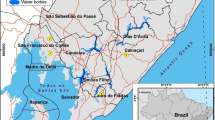
Avoid common mistakes on your manuscript.
1 Introduction
Municipal solid waste management (MSWM) has become a relevant issue worldwide due to the waste generated. In 2016, it was estimated in 2.01 billion tons globally. Latin American and the Caribbean were responsible for 231 million tons, with an average of 0.99 kg/hab/day, expected to increase by more than three times by 2050 (World Bank, 2018). Socio-economic factors have been identified as the main reason for this phenomenon (Kannangara et al., 2018). Solíz Torre (2015) directly relates towns' development and municipal solid waste (MSW) generation. It becomes necessary to work under integrated solid waste management (ISWM) system to tackle MSWM appropriately. It includes principles, activities, policies, and planning to efficiently work with a modern and interconnected framework, where the entire management chain (forecasting, on-site storage, collection, transportation, treatment, and final disposal) is addressed in a sanitary and environmentally friendly manner (Asefi & Lim, 2017a). To be sustainable, it needs to consider economic affordability, environmental effectiveness, and social acceptability to prevent short- and long-term harmful effects on the environment and human health (Khoshbeen et al., 2020).
In Latin America, the sustainable concept is poorly managed through the traditional implementation of a linear economy rather than a circular economy, a still-emerging issue in the region (Betancourt & Zartha, 2020; Diaz, 2017; Dumlao & Halog, 2017). The economic model applied defines ISWM efficiency. Therefore, they face low-financing and weak governance problems. The first one involves a lack of technical personnel and opportunities to apply avant-garde techniques (Ferronato et al., 2019). Meanwhile, the second one refers to the lack of environmental education, inappropriate regulatory and legislative frameworks, and political disinterest (Hettiarachchi et al., 2018). However, Bui et al. (2020) also refer to regional socio-cultural disadvantages. They all agree on the lack of quality information and knowledge for appropriate territorial planning and urbanization processes, impacting on public health (Marshall & Farahbakhsh, 2013), and the environment (Mamashli & Javadian, 2021). Inappropriate and inadequate MSWM increases the difficulties concerning MSW facilities' location regarding public opposition, land cost, and a lack of adequate land.
Within the ISWM system, transfer stations (TSs) emerge, as a sustainable alternative, to optimize the collection-transportation phase, representing between 50 and 70% of the global cost (Rathore & Sarmah, 2019). A TS is a technically established place to allow non-hazardous MSW discharge and temporary storage before the transportation to the final disposal sites (FDSs) (Eiselt & Marianov, 2015; Yadav & Karmakar, 2020). More volumetric capacity vehicles, appropriate for travelling long distances, are used. Hussain et al. (2014), Li and Huang (2010), and Yong et al. (2009) agree that direct transportation is not always appropriate because FDSs are distant from populated areas to prioritize public health (Rathore & Sarmah, 2019). Using TSs presents four advantages: (1) Optimization and efficiency of collection trucks, which results in collection coverage growth. (2) Commonwealth (voluntary associations between different municipalities) formation viability, since small communities do not present the minimum conditions (e.g., resources, inhabitants, MSW generation) to manage an ISWM. Indeed, it will also help to minimize the number of uncontrolled waste disposal areas (Tello et al., 2010). (3) Commonwealth MSW facilities reduce the harmful environmental impacts on residential areas (Bovea et al., 2007). (4) Transportation efficiency by reducing the number of trips to FDSs by using more capacity trucks to cover the route from TSs to FDSs (Bosompem et al., 2016). Thereby, TSs are economically viable and will reduce the collection cost by around 30% (Rathore & Sarmah, 2019). In developing countries, Chile has experienced a reduction of 33.3% (Vásquez, 2005), while Indian a 25% (Paul et al., 2020). A relevant aspect is that TS also reduced energy consumption by 38% and toxic components emission into the air and water due to lower fuel consumption, resulting from fewer trips (Rafiee et al., 2011). The insertion of TSs within ISWM demonstrates profitability (Greco et al., 2015; Lin et al., 2020), effectiveness (Galan et al., 2013), and economic and environmental efficiency (Bovea et al., 2007). To achieve it, TSs need to be correctly located in the territory and looked up holistically, because it is not only based on the costs but also the environmental problems and social affairs, to prevent the occurrence of irreversible consequences (Azadeh et al., 2019).
Vitorino et al. (2017) schematized six methodological categories for MSWM, including mathematical optimization and multi-criteria decision analysis (MCDA). In concordance with them, the literature review exposed in Table 1 briefly describes recent studies about TS's location facility and brings up the same methodologies. The mathematical optimization method aims to solve complex optimization problems by balancing a diversity of ISWM features (Yu & Solvang, 2017), i.e., overall operating costs, time-consuming, risks, environmental impacts, amount of MSW transferred, resources used (Ghiani et al., 2014). On the other hand, MCDA is a heuristic knowledge-based method based on experts' experience (Sellak et al., 2018). Contrary to a cost–benefit analysis done by mathematical optimization models, it is seen as a decision-making framework to analyze qualitative, subjective, and non-monetized social, political, and environmental inputs (Asefi & Lim, 2017a; Cegan et al., 2017; Rathore & Sarmah, 2019). According to Zanghelini et al. (2018), it is defined as a mathematical methodology toolset that combines the technical element with decision-making values to offer: (1) a single favored alternative, (2) a list of ranked alternatives, or (3) acceptable—unacceptable possibilities (Achillas et al., 2013). It considers the problem from its most characteristic variables, analyzed it to achieve a comprehensive solution by quantifying the territory's suitability. Criteria selection for a specific issue and the consideration and flexibility of experts' judgment are key features of this framework.
The optimal location of MSW facilities minimizes used resources in a world known for its space and natural resources limitations. In the face of the environmental problem, it is indispensable to integrate Geographical Information Systems (GIS) capabilities with MCDA methodology simultaneously to configure GIS-MCDA. It is defined as an interactive computer-based system designed to effectively solve geospatial nature issues (Yalew et al., 2016). GIS is considered a time and cost-reducing tool used for collecting, storing, managing, and processing, as well as analyzing, visualizing, and modelling geographically referenced data, which can deal with social, economic, and environmental limitations (Ajay Singh, 2019), appropriate for MSW facilities location issues (Dutta & Goel, 2017). Instead, MCDA provides a vast set of techniques for structuring problems, designing, evaluating, and prioritizing alternatives. GIS-MCDA allows tackling spatial decision-making and territorial assessment problems according to an assortment of criteria and decision-makers preferences, which motivates to increase the number of GIS systems that integrate MCDA techniques.
Since 2000, a rapid increase in published research applying MCDA has been accomplished (Marttunen et al., 2017). Cegan et al. (2017) and Huang et al. (2011) analyze the acceptance of this methodology in environmental science, while Achillas et al. (2013) focus the analysis on its application on MSWM issues, dividing them into "optimal strategy" and "optimal location." In the first category, there is material flow analysis (e.g., Makarichi et al., 2018), MSW collection (e.g., Amal et al., 2020). Optima location has been covered in depth by Farahani et al. (2019) in urban areas where the discussion covers types of decisions, location models, objective function, and constraints. But in an MCDA context, there are a considerable number of studies for MSW facilities location, where the most representative facilities are as follows: landfills (e.g., Cobos & Solano, 2020; Özkan et al., 2020; Randazzo et al., 2018; Yalcinkaya & Kirtiloglu, 2021; Zarin et al., 2021), waste energy plant (e.g., Wu et al., 2016); TSs (e.g., Ağaçsapan & Çabuk, 2020; Asefi & Lim, 2017a, 2017b; Bosompem et al., 2016; Rafiee et al., 2011); construction and demolition dumps (e.g., Santos et al., 2021) and anaerobic digestion plants (e.g., Abdilah, 2020).
Under the exposed, the main aim of this study is to identify optimal TSs locations in the Azuay province of Ecuador based on a territory suitability index (TSI). To the best of the authors' knowledge, no related studies have been conducted in the study area. The authors conceive this research as a locational problem and exclude the routing because it could be studied independently (Araee et al., 2020) due to its different planning periods (Yadav et al., 2016). Location MSW facilities is a significant issue in ISWM due to the system's complexity depends on it. The commonwealth proposal applies a GIS-MCDA with an analytic hierarchy process (AHP) technique. The authors approached the problem holistically from economic, technical, environmental, and social criteria.
2 Material and methods
2.1 Study area
The province of Azuay in Ecuador is located in the Andes mountains of South America, and it is part of the Latin America community. Politically-administratively, it is divided into 15 cantons, Cuenca, Girón, Gualaceo, Nabón, Paute, Pucará, San Fernando, Santa Isabel, Sigsig, Oña, Chordeleg, El Pan, Sevilla de Oro, Guachapala and Camilo Ponce Enríquez. According to the territorial organization, autonomy and decentralization organic code, Article 55, paragraph d) (National Assembly of the Republic of Ecuador, 2010), cantonal governments are responsible for ISWM.
The last official population census conducted in Ecuador in 2010 (National Institute of Statistics & Censuses of Ecuador, 2012) showed a particularity in Azuay province. Cuenca canton has 70,1% of the total inhabitants, with the region's second-highest growing index (0.018%) less than Camilo Ponce Enriquez (0.046%). Calculated data for 2018 shown an urban per capita of 0.56 for Cuenca, which is not the highest because Sevilla de Oro´s is 0.84. Azuay province has two FDSs, Pichacay, which receives MSW from six cantons as a lease, and Huscachaca, which serves four cantons joined in the Jubones watershed commonwealth. The other cantons leave their MSW in open dumps or landfills outside the province. On regard to distance traveled from generating nodes to FDSs, the longest routes are those coming from Nabón, Sevilla de Oro, Pucará, El Pan, Guachapala, and Gualaceo. The shortest distances have those cantons with an FDS under their political administrations. Nowadays, Azuay does not have implemented TSs as part of its ISWM system (Table 2). The model notation that takes place in this research is shown in Table 3.
2.2 Analytical Hierarchical Process (AHP) as MCDA technique
The AHP proposed by Saaty (1980) is based on the criterion pairwise comparison matrix A with n x n dimensions. To implement this process, it is necessary to (1) define the problem to be analyzed; (2) analyze the problem according to its variables; (3) rank options by creating pairwise comparison matrices; and (4) obtain the sensitivity or feasibility analysis' results (Veitia et al., 2014). Criteria pairwise comparison utilizes a numerical scale ranging from 1 to 9, where 1 represents equal importance between the compared pairs, and 9 represents extreme importance of one criteria over other (Saaty, 1994). The values must be added above the leading diagonal as the cells below will be completed applying the reciprocity axiom according to Eq. (1).
The normalized matrix N is calculated from matrix A according to Eq. (2), which averages for each row results in the weighting vector (W). An essential characteristic of this method is the experts' judgment evaluation by the consistency ratio (CR) through Eq. (3) (Karim Ghani et al., 2013). CR must be less than 0.10 (10%) to suggest a consistent matrix A. If this ratio has a higher value, then matrix A needs to be reconsidered. For calculating CR, the consistency index (CI) must be previously calculated with Eq. (4), the random index (RI) according to Eq. (5), and \(\lambda max\) with Eq. (6).
Professional and qualified experts for pairwise comparison matrices are essential; they can consider the weaknesses and strengths of each criterion and manage them consequently. However, their judgment adds uncertainty, which can be reduced in the decision-making procedure by including more standards in the analysis. This technique could merge various types of stakeholders. For example, Kamdar et al. (2019) used ten experts conformed by public administrators, environmental agents, and other professionals in civil engineering, soil science, mining and materials engineering, sociology, and waste-to-energy gasification operations. Kahraman et al. (2018) used three experts who represent government, academic and non-profit organizations. Solangi et al. (2018) consider five experts classified into four types academics, energy, economics, and other stakeholders. The number of experts depends on each decision problem, where it is necessary to consider the resources available and the perceived level of importance of the decision (Hyde et al., 2003). This research has five experts: two academics in geographical and environmental science (one of each), one civil engineer, and two representatives of MSWM public administrations and field experts. Besides, social representatives were also included. All of them were familiar with the local situation and needs.
This research uses a three-level hierarchical schema shown in Fig. 1. It starts from a general perspective to a specific one. In the first level, to commune with a sustainable ISWM system and concordance with Araiza Aguilar et al. (2018) and Yadav et al. (2020), environmental, social, economic, and technical aspects were used. The second level consists of criteria related to each specific category, and the third level involves criteria´s measurable attributes. This hierarchy differs from what is done by Khan et al. (2018), who only uses a two-level analysis with criteria and sub-criteria weighting. The criteria used in this study for TSs location are shown in Fig. 1a, and the restrictions, which nullify the possibility of TSs location, are shown in Fig. 1b. The proposed methodology differs from other TSs location researches, where TSs are analyzed from an economic optimization model (e.g., Asefi & Lim, 2017b; Li et al., 2019; Shyamali & Kumar, 2021).
The AHP process is appropriate for categorizing, criteria', and sub-criteria weighting. In this study, each category was analyzed with its corresponding criteria. A simple additive weighting was applied to obtain TSI for TS (Sahir et al., 2017). The result will be a raster coverage gotten by Eq. (7); then, it is essential to spatially subtract the restrictions by Eq. (8). The higher the TSI values, the more appropriate the territory is, which is given as follows:
2.3 Gravity center (GC) calculation
The GC concept is derived from physics; however, geography indicates the variable's spatial equilibrium point in a given area (Zhang et al., 2012). GC helps to understand spatial–temporal dynamics in a more precise manner. Therefore, it has been used in population distribution analysis (Kumler & Goodchild, 1992), urban expansion (H. Wang, Zhang, et al., 2020; Wang, Zhao, et al., 2020), and MSW generation (K. Wang et al., 2020; Wang, Zhao, et al., 2020). For TSs' location, GCs have been considered under three adjustment variables: population density, per capita generation, and the total amount of daily generated MSW. It is important to emphasize that the higher the population density, the greater the amount of generated MSW (Pan et al., 2019); therefore, it demands greater ISWM efficiency. The purpose of CG is to place TS as close as possible to higher demanded MSW collecting areas (Pluskal et al., 2020).
These three adjustment variables must be projected to the infrastructure design period, Dai et al. (2011) formulate five years, Kirca and Erkip (1988) ten years, Tan et al., (2010a, 2010b) fifteen years, Appel et al. (2018) twenty years, and Galante et al. (2010) a thirteen years lifespan. These periods may vary according to the limitations and capacities of the study area; consequently, the authors decided on a twenty-five-year lifespan. The projections must start from official data, although it is acknowledged that not all countries, especially Latin Americans, have complete, updated, and accurate information; therefore, mathematical and statistical calculations can be used to fill the gaps.
The population attended at the end of the lifespan was calculated using the exponential projection by Eq. (9). From the projected population served, population density at the end of lifespan was calculated using Eq. (10).
Daily MSW per capita generation projection (dpgp) is calculated with Eq. (11), where the value 0.7% is a constant established by Collazos and Duque (1993) for annual per capita growth.
MSW's collection coverage projection expressed in percentage (Ccp) is calculated using Eq. (12), where 1.27 is the annual coverage growth according to Collazos and Duque (1993). With known CCp and dpgp, the total MSW generated daily (Aswg) is calculated according to Eq. (13).
After calculating the adjustment variables and separating them according to the lifespan, a quadrangular mesh was generated ("create fishnet" tool, in the Arcgis software) to calculate GCs coordinates according to Eq. (14) and Eq. (15) (Oktrianto & Siswanto, 2017). All three GCs for each administrative boundary (canton) are reduced to one by finding a geometric center; this last one is called integral cantonal GC.
2.4 Validation model
The MCDA model depends on the weights extracted through the AHP technique, introducing the expert´s subjectivity through the pairwise comparison matrix. To understand those facts, a sensitivity analysis has been used. One of its aims pretends to validate the effect on the outputs (final raster) by changing the inputs parameters (criteria weighting) (Maliene et al., 2018). The desire is to have models less sensitive to changes because if the change of a specific criterion results in a relatively significant output change; it implies that the model depends on that criterion (Feizizadeh & Blaschke, 2014). Leonelli (2012) divides the sensitivity analysis into three categories (1) numerical incremental analysis, known as one-at-a-time. It is the most common method. This approach has been used in Ajjur and Mogheir (2020), Alemdar et al. (2020), Ghajari et al. (2017), Madruga de Brito et al. (2019). (2) Probabilistic simulations which use the computer to replace judgments according to probability distributions. It needs to be performed a vast number of times as the Monte Carlo simulation. This approach has been used in Mohamadzadeh et al. (2020) and Feizizadeh et al. (2021); (3) Mathematical models which expressed the relationship between inputs and the solution. The first technique is assumed in this research as a validation method, where 26 interactions were run, building the same number of scenarios, each one modifies a criterion´s weighing while the rest remain the same (the weighting of categories and sub-criteria do not change in any scenario). Scenarios 1 to 13 were added 10% to the original criterion´s weighting, and scenarios 14 to 26 were subtracted 10% as shown in Table 4.
3 Results
3.1 Criteria for TSs location: a bibliographic review
A bibliographic review helped to select the TS location criteria. The searching keywords used were sanitary landfills, TSs, and MSW facilities in general, owing to some criteria playing similar roles in those infrastructures. However, the study area has not presented scientific-related studies; thus, the review had to extend to 28 scientific manuscripts from different geographical contexts (Habibi et al., 2017; Hariz et al., 2017; Josimović et al., 2015; Khadivi & Ghomi, 2012; Kumar et al., 2020; Liu et al., 2018; Roé et al., 2014; Shi et al., 2019; Tavares et al., 2011; Thampi & Rao, 2015; Yalcinkaya, 2020; Cobos et al., 2017; Moeinaddini et al., 2010; Rahimi et al., 2020; Singh & Vidyarthi, 2008; Bosompem et al., 2016; Chang and Lin, 1997; Chatzouridis & Komilis, 2012; Gil & Kellerman, 1993; Jaiswal & Bharat, 2016; Mantzaras & Voudrias, 2017; Perdomo & Ramirez, 2011; Rafiee et al., 2011; Rathore & Sarmah, 2019; Varón et al., 2015; Yadav et al., 2016, 2018, 2020), eight technical studies (Ministry of Environment & Natural Resources of Mexico, 2010; National Institute of Preinvestment of Ecuador, 2013; Waste Agency of Catalan, 2016; González, 2010; García, 2008; Municipal Public Cleaning Company of Cuenca (EMAC-EP), 2006; Sánchez & Estrada, 1996; Secretariat of Social Development of Mexico, 2001); ten theses (Amutio, 2019; Erazo & Villaroel, 2015; Hernández & Rodríguez, 2016; Massukado, 2004; Medina, 2009; Merchán & Pomavilla, 2019; Ortega & Torres, 2016; Ribas, 2019; Tapia, 2008; Urriago, 2018); and seven Latin American environmental regulations regarding MSWM (Ministry of Human Development of Bolivia, 1996; Ministry of the Environment of Ecuador, 2010; Ministry of the Environment of Guatemala, 2016; Presidency of the Republic of Costa Rica, 2014; Presidency of the Republic of Nicaragua, 2002; Ministry of the Environment of Peru, 2017; Presidency of the Republic of El Salvador, 2002).
Thirty-four criteria were evidenced such as (they are named in order to the highest to the lowest frequency): distance from roads (A), distance from populated areas (B), distance from rivers (C), distance from protected natural areas (D), slopes (E), distance from generating nodes (F), unstable zones (G), land use (H), geology (I), basic services availability (J), weather (K), distance from the power grid (L), transportation costs (M), distance from tourist sites and churches (N), type of property (O), amount of MSW generated (P), public health effects (Q), groundwater (R), distance from health centers (S), water bodies (T), airports (U), distance from educational centers (V), distance to landfills (W), hydrocarbon or conduction infrastructure (X), job opportunities (Y), elevation (Z), soil permeability (Aa), waste type (Ab), public acceptability (Ac), child population density (Ad), coverture level (Ae), industrial areas (Af), soil compaction factor (Ag), and administrative boundaries (Ah). The six first outstanding criteria strongly marked the economic and socio-environmental significance. On the other hand, there are unpopular criteria considered in less than 5% of the documentation reviewed, as the last eleven criteria (Fig. 2).
The analysis grouped specific criteria into categories: urban and rural areas, called "populated areas." Natural, anthropic, and culturally valuable areas are classified as "protected areas." Seismic, geological faults, flood-prone zones (alluvial plains), and landslides are conceived as "unstable areas." Temperature, humidity, precipitation, and wind correspond to the "weather" group. Gases, odour, and noise are grouped as "public health effects." The mangroves, swamps, wetlands, estuaries, and aquifers are classified as "water bodies." Finally, the pipelines, gas stations, and factories are part of the "hydrocarbon or conduction infrastructure."
3.2 GCs on the study area
To calculate GCs was necessary to project adjustment variables until lifespan (Table 5). In 2045, Cuenca will be the central MSW generator node representing 72,3% of MSW with 972,184 inhabitants and a population density of 305 hab/km2, the region's highest density. However, with 0,68%, this city will not have the most increased urban per capita generation because other cantons like Sevilla de Oro reach 1.01 kg/hab/day. On the other hand, Chordeleg, Sevilla de Oro, Oña, Paute, Santa Isabel, Guachapala, Nabón, San Fernando, Girón, El Pan, and Pucará, produce less than 20 t/day each. This amount is not representative to manage ISWM systems individually in terms of cost, environmental impacts, and administrative capacities, justifying the commonwealth's formation.
According to the adjustment variables for each administrative division, the three GCs are in the cantonal populated area or close to them; consequently, the integral cantonal GCs do so. Figure 3 shows each GC and the provincial GC (redpoint), close to Cuenca due to its particularities already discussed.
3.3 Criteria weighting according to the AHP technique
Table 6 shows the categories, criteria, and sub-criteria weighting, with restrictive sub-criteria. According to these results, the technical class leads the process, followed by environmental, social, and economic categories, respectively. As the pairwise comparison matrix was built individually for each category, the most significant criterion was the slope in the technical category, while the distance from power grids was the least important. In the environmental category, the most relevant criterion is the distance from rivers, and the distance from populated areas is the least significant. The highest weighting corresponds to land use in the social category and the lowest to distance from tourist sites. The distance from GCs has the highest weighting in the environmental category, and the availability of basic services has the lowest.
3.4 Application of GIS-MCDA at Azuay province
A maximum TSI of 89% and a minimum of 13% were obtained after applying GIS-MCDA over 13 criteria, using simple additive weighting and subtracting the restrictive variables (Fig. 4). According to the analyzed criteria and expert's judgment, it is evident that the study area does not have a perfect site (TSI = 100%) for TSs' location. The area categorized as restrictive occupies most of the provincial territory with an area of 6,602.94 km2 out of 8,325.16 km2, representing 79.31%. Thus, more than 90% of Pucará, Guachapala, Chordeleg, and El Pan cantons are not suitable for TSs location. On the other hand, Paute, Santa Isabel, Girón, and San Fernando present more suitable territories under their administrations (Fig. 5a).
The Azuay suitable area represents 20.69%. The distribution at the cantonal level, categorized by TSI, is detailed in Fig. 5b. The highest TSI stands between 70 and 89%. It represents just 0.29% (23,82 km2) of the entire province. The intermediate category, standing between 50 and 70%, corresponds to 5.5% (457,87 km2). Finally, the lowest category, between 13 and 50%, occupies 14.90% (1,240.53 km2). When trying to find optimal locations, it is not possible to pick up areas with one specific TSI category or value. Thus, the authors localize the highest values and analyze them with the surrounding areas to form the most convenient polygon according to the study area´s reality. In this context, cantons with higher territorial extension to locate TSs are Cuenca, Santa Isabel, Sigsig, Nabon.
Based on the previous information, three TSs potential sites were established for nine of the fifteen cantons (Fig. 4). TS 1, located in Paute, will be used by this canton, Sevilla de Oro, El Pan, and Guachapala. TS 2, located in Sigsig, will be used by this canton, Chordeleg and Gualaceo, where Pichacay is the FDS for both cases. Nabón and Oña will constitute TS 3. It will be placed in the first canton, and Huascachaca will be used as FDS. Girón, San Fernando, Pucará, Santa Isabel y Cuenca will not use any TS.
This proposal shows a significant improvement in the distance comparison with and without TS between the nine cantons shown in Table 7. The distance travelled by Nabón will reduce 41,6 km. Paute will not shorten its length because it's being used as an FDS within the administrative boundaries in an emerging cell with a lifespan that is about to end. However, this proposal uses this site as TS 1 and suggests that Pichacay should be the FDS for Paute. Some cantons do not reduce their travelling distance. Still, it is necessary to consider other factors such as cost optimization, which can be achieved by reducing the number of trips to FDSs by using higher capacity vehicles. It is important to consider that some small cantons do not justify daily trips with only their MSW, which also implies an environmental improvement.
3.5 Validation model results
Table 8 shows the results of each scenario run in the sensitivity analysis. It represents the percentage of the area changed (according to the original area) in each scenario, categorized by TSI. The most significant area change occurred in three scenarios: (1) The thirteenth where the slope´s weighting incremented by 10%, the area´s change was 0,51%. (2) The fourteenth scenario changed by 0,42% of its area, and it represents the subtraction by 10% over the distance from rivers weighting criterion. (3) The last significant change in area with a 0,38% occurred in the twenty-sixth scenario, where the slope also changed. This time, 10% was subtracted from its original weighting. Based on these results, the authors conclude that the overall sensitivity of the presented model is inferior to 1%, which shows that there are no critical criteria; besides, its outputs are robust and reliable.
4 Discussion
4.1 Relevance of TS location categories and criteria
The optimization models consider TSs location as one of the multiple objectives to solve, additionally to TSs number (Yadav et al., 2016), MSW type (Asefi et al., 2015), routing (Asefi & Lim, 2017b), overall cost-minimizing (Eiselt & Marianov, 2014), TS capacity (Kůdela et al., 2019), time optimization (Monzambe et al., 2021) amount others. From those perspectives, it is impossible to analyze in detail the terrain suitability to host a TS based on environmental, social, economic, and technical variables and their interactions. For the knowledge of the authors, there has not been published TSs location variables review. Consequently, the literature review from scientific studies, technical documents, thesis, and environmental policies, presented in Sect. 3.1, supplies by itself a contribution for MSWM decision-makers and further studies.
The holistic perséctive of this research considers four categories: The World Commission on Environment and Development (1987) and the United Nations General Assembly(2005) stated the environmental, social, and economic categories as fundamental for sustainability and development. The technical category was incorporated in MSW management, attending to its characteristics. All categories have been analyzed together in the MSW field by Son and Louati (2016), Turcott Cervantes et al. (2018), and Vucijak et al. (2015).
The technical category is relevant because it influences the facility's stability. Regarding this, the corresponding criteria are geology, distance from power grids, and slopes. In terms of geology, the ideal soils are rocky with a high bearing capacity, and they generally do not present landslide risks (Rahimi et al., 2020). However, silty-loam or silty-clay soils have been suggested as those with the best conditions for Andean reality (Cobos & Solano, 2020). A distance from power grids must be considered because it should not mean difficulty for TSs operation, nor be opposed to its power lines. The Ministry of Human Development of Bolivia (1996) recommends a minimum 300 m buffer zone, while the National Institute of Preinvestment of Ecuador (2013) considers it should be 100 m. In contrast, Yalcinkaya (2020) suggests that TS should not be placed further than 1000 m around power lines to avoid access costs. Finally, land slopes are important to create access (Rahimi et al., 2020). Also, it is advisable to avoid steep slopes to reduce construction costs and ease the vehicles' movement and manoeuvring. The optimal slopes should not exceed 9% (Ağaçsapan & Çabuk, 2020).
The contemplation of an environmental category pretends to minimize the TSs operation negative impacts on the ecosystem. Bovea et al. (2007) stated a proportional environmental impact concerning the distance and amount of MSW transported based on a life-cycle assessment technique application. In concordance with Bosompem et al. (2016), the distance from rivers has been stated as the most significant criterion. Contrasting FDS, this criterion evaluation took place under the assumption that contamination is coming from cleaning tasks rather than leachate percolation. Although the TS must be built so that technically elaborated drainage systems evacuate all generated fluids, there is always the possibility of unexpected spills inside or outside the infrastructure. Besides, the Ministry of the Environment of Ecuador (2010) and Roé et al. (2014) consider a restrictive protection zone from 200 to 500 m, respectively, from water bodies. The protected natural areas are considered fragile ecosystems, which are vulnerable to the adverse effects of changing ecosystems that result from significant environmental deterioration caused by pesticides such as organochlorine, acidification, and eutrophication coming from TSs (Sultan et al., 2019). According to the Ministry of the Environment of Ecuador (2010), these facilities should not be located less than 500 m from these areas to protect them from anthropic intervention, while Rahimi et al. (2020) propose a 1000 m radius. Finally, distance to populated areas was also considered. Its purpose was to protect public health from TSs adverse effects such as bacteria that could be spread out with the wind (Hasbiah et al., 2021), noise, gas emissions, and odors (Sun et al., 2017). Chang et al. (2019) establish a 500 m restrictive area when the smell is intensely concentrated. Volatile organic compounds in a TS could have potentially severe and chronic health risks (J. Liu & Zheng, 2020; Sarkhosh et al., 2017). All environmental criteria are evaluated in a way to prioritize the furthest areas from them.
According to the inhabitants' perception, TSs are harmful environmental facilities because of most of the aforementioned ecological implications. They categorized them with the not-in-my-backyard syndrome, known as NIMBY, as Zhang et al. (2018) discussed. The social perspective is mainly oriented to minimize the possible opposition caused by this syndrome and protect public health. However, Cirucci et al. (2015) also use related criteria in this category to evaluate MSW generation. Even though this research does not analyze TS's social perception and acceptance, the authors address related standards to minimize opposition by contemplating and safeguarding relevant aspects such as land use, social facilities such as education, health and tourist centers. Regarding land use, it should preferably be intervened in bare areas or grassland; however, according to Saavedra and Nogales (1998), the sites with less than 40% of vegetation can also be helpful. The focus here is to protect the forest, aerial, and agricultural land from anthropic degeneration (Khan et al., 2018) because Lella et al. (2017) identify an annual reduction of 2,100 m2 of vegetation cover around the MSW facility. In addition to protecting biodiversity, the purpose of this criterion is to protect the municipal principal economic activity. Urban planning is essential since it organizes a society, considering the current use of land and how it can be used without harming and risking citizens' security. According to social infrastructure, it is necessary to restrict buffer zones. The Ministry of Environment and Natural Resources of Mexico (2010), Erazo and Villaroel (2015), and Kumar et al. (2020) suggest 500 m. Besides, the effectiveness of a health center is essential for developing emerging economies. The visual impacts, aesthetic and progressive deterioration or abandonment of areas around MSW facilities represent a problem for tourist sites. From the last three criteria, TSs must be located as far as possible. There is a 13 km and 500 m restrictive buffer from airports and archaeological, respectively.
The economic aspect plays a vital role in ISWM system efficiency. Most researchers in this field conceive this category under cost assumption. Thus, Kůdela et al. (2019) incorporate the transfer cost with and without TS, construction, processing, operation, and maintenance. Conjointly with travel's distance and time which can be quantified through cost as well. This contrast with Höke and Yalcinkaya (2021), where a quantitative economic assessment is carried out by fuel, vehicle, and labour costs because the siting and routing issues are joined analyzes. This work focuses on terrain suitability and implicitly addressed the price according to basic services availability, distance from roads, and distance from GCs. According to the first criterion, Yadav et al. (2020) emphasize water and electrical services, while Hariz et al. (2017) include health services. For the study area, in addition to those already indicated, The National Institute of Statistics and Censuses of Ecuador (2020) considered drinking water, sewerage, phone service, and garbage collection. The purpose of the distance from roads criterion is to avoid new road designing. However, due to the heavy traffic at TSs, they must have a 200 m (Ministry of the Environment of Ecuador, 2010) to 500 m protected zone around (Mantzaras & Voudrias, 2017). According to legal speed limitations, this research leaves apart roads classification as it is considered on Ağaçsapan and Çabuk (2020) because Mohsenizadeh et al. (2020) demonstrates that truck's velocity has no implications in cost reduction. Besides, it differs from Louati et al. (2019) and Yadav et al. (2017), which integrated a vehicle routing analysis as an optimization parameter for an ISWM. Concerning distance from GCs, this study rewards those areas between 0 and 20 km (first two sub-criteria) with the highest weight because Kaghembega et al. (2020) established that TSs must be as close as possible from generating nodes. Rathore and Sarmah (2019) reveal a transportation cost reduction by using a TS when the distance between FDS and generating nodes is higher than 15 km. The proposed GCs spatial calculation marks a substantial improvement about Bosompem et al. (2016), Chang and Lin (1997), Jaiswal and Bharat (2016), Perdomo and Ramirez (2011), Rafiee et al. (2011), and Varón et al. (2015), where generating nodes are considered, which can be urban areas perse or its centroid. Here, GCs are equilibrium points based on population density, daily generation, and per capita generation, fundamental variables in MSW analysis.
A provincial GC was also calculated but due Cuenca is and will be the main MSW generating node by far from other cantons, it falls into Cuenca or its surroundings. Its use would pull TSs to Cuenca and will limit an integral solution minimizing small cantons' needs. Thus, cantonal GCs were considered in the GIS-MCDA.
4.2 Relevance for the province of Azuay
This proposal is based on the real needs of the study area, where a single canton—Cuenca—is consecrated as the more significant MSW generator, but this will remain until 2045. Nowadays, Cuenca's ISWM is the most efficient and sustainable of the province. On the other hand, small cantons (e. g., Generate less than 20 tons) with insufficient technical, economic, and administrative resources also exceed the direct transportation recommended distance. Most of them have undertaken commonwealth or lease an FDS as alternatives. The last one comes to be an easy solution because it causes municipalities to ignore their environmental and social responsibilities. Instead, commonwealth formation is based on Article 243 of the Republic of Ecuador Constitution (Constituent Assembly of Ecuador, 2008) and promoted by the Ecuadorian Ministry of the Environment, Water and Ecological Transition, to the point to have 23 commonwealths created in 2016 (Siclari & Olivotto, 2021). This concept arose in Europe in countries like Spain, Italy, and France. These associations are efficient and demonstrate a useful organizational and economically tool to solve issues that do not respect territorial boundaries (Gough & Accordino, 2013). In MSWM, commonwealth denoted substantially improved services, with lower investments for each municipality and more significant benefits (Vega, 2016) such as: to improve the ISWM system in small cantons by reduction of global and individual cost, optimization and strengthening installed capacities, generation of responsibility and transparent culture, enhance the quality of service, autonomy management, centralization of MSWM, which favors the operation and reduces the environmental impact (Cobos et al., 2017). Finally, three TSs were proposed to integrated nine cantons: Paute, Sevilla de Oro, El Pan, Guachapala, Sigsig, Chordeleg, Gualaceo, Nabón, and Oña. However, the remaining cantons have different realities which should be carefully studied independently. For example, Cuenca's high amount of MSW needs a local TS also used as a green point, but the analysis conditions differ from what has been approached here because it needs to be in an urban area. Girón, San Fernando, Pucara and Santa Isabel are close to the FDS. However, the first three do not have enough MSW for individual trips. Camilo Ponce Enriquez presents geographic and communication characteristics that challenge annexing it to any proposed TS or existing FDS within the province. Therefore, it is advisable to strengthen its own FDS under quality and environmental standards or join another province with more suitable conditions.
Within the ISWM system, TS has gone from being optional to being a fundamental part of the transport phase. Nevertheless, except for Peru (Ministry of the Environment of Peru, 2017) and El Salvador (Presidency of the Republic of El Salvador, 2002), Latin American legal codes have not included regulations for their correct implementation. Regarding MSW facilities' location, the Ministry of the Environment of Ecuador (2010) gives some technical references to implement FDSs, focusing mainly on restrictions. However, for TS, they only state the necessity to implemented under environmental authority permits. It leaves a critical legal gap and needs to create technical norms starting from the basis (technical, scientific, and academic staff) upwards (legislators). Furthermore, it is crucial to considered citizen education about MSW source separation and recycling to increase socio-environmental benefits and reducing the operational cost of doing it in TSs (Mofid-Nakhaee et al., 2020). Although this last aspect is covered by the Ecuadorian Organic Environment Code (Ministry of the Environment of Ecuador, 2017), as a principal, there is no legal instrument for regulation and control. On a national level, its fulfilment achieves only 36.4% (National Institute of Statistics & Censuses of Ecuador, 2020).
5 Conclusions
The main goal of this research was to find suitable locations for TSs in the province of Azuay in Ecuador. To achieve it, the authors formulate a three-level hierarchy structure. The categories, criteria and sub-criteria were evaluated according to a provincial reality, which can be discussed differently for other contexts. However, this study proposes a methodological guide to help local governments and decision-makers to build their policies and regulations regarding MSW transportation phases. Even more with oversimple ISWM system regulations and lack of technical norms experienced in Latin American countries. A regional approach by a commonwealth formation is recommended. Besides, the work-scale for the geographical context became an alternative to propose a middle-scale map by first diagnosing the entire area. Later efforts must concentrate on the potential sites presented here by in situ inspections and studies such as optimal routing modelling, environmental impacts, and social conflicts. In order to deal with public opposition, not considered in this research, it is necessary to undertake a participatory methodological process, where political commitment, citizen opinion by a group of interest, and the community's education are highly valued.
The authors implemented the AHP technique using the GIS-MCDA methodology, widely used for site location issues because of its low cost and easy-to-use. It converts complex decision-making issues into simpler hierarchal and pairwise analyzed systems. It allows the easy inclusion or elimination of criteria, making it scalable and providing different scenarios by weighting recalculation. In the process, it builds a geographical database with qualitative and quantitative attributes for a monitoring process over time. The popularity of this integrated framework has been extended because of scientific world acceptance and the computer science improvement to process spatial data. However, the optimization perspective from which TSs are approached to handle MSW transportation has focused the researchers' interest on the economic factor, forgetting environmental, social, and technical aspects to define the suitability of the terrain. The latter gives to this research the appeal by addressing an underrated issue. This methodology provides a flexible tool about the number of criteria, formats, scales, and sources of how the geo-information is represented.
Finally, for future researches, the entropy of the uncertainty environment introduced by the ambiguity, vagueness, and inaccuracy of experts' judgment can be better handled by fuzzy sets theory, widely used on site-location problems. If the problem warrants it, an analysis of forgotten effects could be added, followed by a contra-expertise procedure to validate the expert's judgment. This process will allow recovering the expert omissions incidence by validating the evaluators' subjectivity to improve decision-making process quality. Additionally, a probabilistic sensitivity test as Monte Carlo analysis could be carried out as a validation model.
Availability of data and material
Not applicable.
Code availability
Not applicable.
Change history
03 March 2022
A Correction to this paper has been published: https://doi.org/10.1007/s10668-022-02220-x
References
Abdilah, F. (2020). Evaluation of potential anaerobic digestion facilities in Selangor, Malaysia by integrating GIS and Multi-criteria Decision Aid (MCDA). GeoSciences MSc Thesis Collection. https: //hdl.handle.net/1842/37643
Achillas, C., Moussiopoulos, N., Karagiannidis, A., Banias, G., & Perkoulidis, G. (2013). The use of multi-criteria decision analysis to tackle waste management problems: A literature review. Waste Management and Research, 31, 115–129. https://doi.org/10.1177/0734242X12470203
Ağaçsapan, B., & Çabuk, S. N. (2020). Determination of suitable waste transfer station areas for sustainable territories: Eskisehir case. Sustainable Cities and Society. https://doi.org/10.1016/j.scs.2019.101829
Ajjur, S. B., & Mogheir, Y. K. (2020). Flood hazard mapping using a multi-criteria decision analysis and GIS (case study Gaza Governorate, Palestine). Arabian Journal of Geosciences, 13, 2. https://doi.org/10.1007/s12517-019-5024-6
Alemdar, K. D., Kaya, Ö., & Çodur, M. Y. (2020). A GIS and microsimulation-based MCDA approach for evaluation of pedestrian crossings. Accident Analysis and Prevention. https://doi.org/10.1016/j.aap.2020.105771
Amal, L., Son, L. H., Chabchoub, H., & Lahiani, H. (2020). Analysis of municipal solid waste collection using GIS and multi-criteria decision aid. Applied Geomatics, 12, 193–208. https://doi.org/10.1007/s12518-019-00291-6
Amutio, C. (2019). Diseño de una planta de valorización de residuos urbanos para el área de gestión A6 de la Comunitat Valenciana. Universidad Politécnica de Valencia.
Appel, D., Paula, A., Luis, D. C., Amador, M., & Kamila, D. S. (2018). Use of a geographic information system to find areas for locating of municipal solid waste management facilities. Waste Management, 77, 500–515. https://doi.org/10.1016/j.wasman.2018.04.036
Araee, E., Manavizadeh, N., & Aghamohammadi Bosjin, S. (2020). Designing a multi-objective model for a hazardous waste routing problem considering flexibility of routes and social effects. Journal of Industrial and Production Engineering, 37, 33–45. https://doi.org/10.1080/21681015.2020.1727970
Araiza Aguilar, J. A., Nájera Aguilar, H. A., Gutiérrez Hernandez, R. F., & Rojas Valencia, M. N. (2018). Emplacement of solid waste management infrastructure for the Frailesca Region, Chiapas, México, using GIS tools. Egyptian Journal of Remote Sensing and Space Science, 21, 391–399. https://doi.org/10.1016/j.ejrs.2018.01.004
Asefi, H., Lim, S. (2017). A bi-objective optimization approach to a municipal solid waste management system. 15th International Conference on Environmental Science and Technology.
Asefi, H., & Lim, S. (2017). A novel multi-dimensional modeling approach to integrated municipal solid waste management. Journal of Cleaner Production, 166, 1131–1143. https://doi.org/10.1016/j.jclepro.2017.08.061
Asefi, H., Lim, S., & Maghrebi, M. (2015). A mathematical model for the municipal solid waste location-routing problem with intermediate transfer stations. Australasian Journal of Information Systems, 19, S21–S35. https://doi.org/10.3127/ajis.v19i0.1151
Azadeh, A., Ahmadzadeh, K., & Eslami, H. (2019). Location optimization of municipal solid waste considering health, safety, environmental, and economic factors. Journal of Environmental Planning and Management, 62, 1185–1204. https://doi.org/10.1080/09640568.2018.1482200
Betancourt, C., & Zartha, J. (2020). Circular economy in Latin America: A systematic literature review. Business Strategy and the Environment, 29, 2479–2497. https://doi.org/10.1002/bse.2515
Bosompem, C., Stemn, E., & Fei-Baffoe, B. (2016). Multi-criteria GIS-based siting of transfer station for municipal solid waste: The case of Kumasi Metropolitan Area, Ghana. Waste Management and Research, 34, 1054–1063. https://doi.org/10.1177/0734242X16658363
Bovea, M. D., Powell, J. C., Gallardo, A., & Capuz-Rizo, S. F. (2007). The role played by environmental factors in the integration of a transfer station in a municipal solid waste management system. Waste Management, 27, 545–553. https://doi.org/10.1016/j.wasman.2006.03.020
Bui, T. D., Tsai, F. M., Tseng, M. L., & Ali, M. D. H. (2020). Identifying sustainable solid waste management barriers in practice using the fuzzy Delphi method. Resources, Conservation and Recycling, 154, 104625. https://doi.org/10.1016/j.resconrec.2019.104625
Cegan, J. C., Filion, A. M., Keisler, J. M., & Linkov, I. (2017). Trends and applications of multi-criteria decision analysis in environmental sciences: Literature review. Environment Systems and Decisions, 37, 123–133. https://doi.org/10.1007/s10669-017-9642-9
Chang, N. & Lin, Y. T. (1997). Optimal siting of transfer station locations in a metropolitan solid waste management system. Spectroscopy Letters, 30, 601–623. https://doi.org/10.1080/00387019708006686
Chang, H., Zhao, Y., Tan, H., Liu, Y., Lu, W., & Wang, H. (2019). Parameter sensitivity to concentrations and transport distance of odorous compounds from solid waste facilities. Science of the Total Environment, 651, 2158–2165. https://doi.org/10.1016/j.scitotenv.2018.10.134
Chatzouridis, C., & Komilis, D. (2012). A methodology to optimally site and design municipal solid waste transfer stations using binary programming. Resources, Conservation and Recycling, 60, 89–98. https://doi.org/10.1016/j.resconrec.2011.12.004
Cirucci, J. F., Miller, D. A., & Blanford, J. I. (2015). Retrospective GIS-based multi-criteria decision analysis: A case study of California waste transfer station siting decisions. Proceedings of the International Symposium on Sustainable Systems and Technologies. https://doi.org/10.6084/m9.figshare.1512514
Cobos, S., Solano, J., Vera, A., Monge, J. (2017). Análisis multicriterio basado en GIS para identificar potenciales áreas de emplazamiento de un relleno sanitario mancomunado en la provincia del Azuay. XVI Conferencia Iberoamericana de Sistemas de Información Geográfica (CONFIBSIG), 12.
Cobos, S., & Solano, J. (2020). Sanitary landfill site selection using multi-criteria decision analysis and analytical hierarchy process : A case study in Azuay province. Ecuador. Waste Management & Research. https://doi.org/10.1177/0734242X20932213
Collazos H, Duque R, (1993). Residuos sólidos (FUNPIRS). ed. Santa Fe de Bogotá - Colombia.
Constituent Assembly of Ecuador, 2008. Republic of Ecuador Constitution (Issue 20 de octubre).
Dai, C., Li, Y. P., & Huang, G. H. (2011). A two-stage support-vector-regression optimization model for municipal solid waste management e A case study of Beijing, China. Journal of Environmental Management, 92, 3023–3037. https://doi.org/10.1016/j.jenvman.2011.06.038
Diaz, L. F. (2017). Waste management in developing countries and the circular economy. Waste Management and Research, 35, 1–2. https://doi.org/10.1177/0734242X16681406
Dumlao, M., & Halog, A. (2017). Moving towards a circular economy in solid waste management: Concepts and practices. In S. Goel (Ed.), Advances in solid and hazardous waste management (pp. 29–49). Berlin: Springer. https://doi.org/10.1007/978-3-319-57076-1
Dutta, D., & Goel, S. (2017). Applications of remote sensing and GIS in solid waste management – A review. In S. Goel (Ed.), Advances in solid and hazardous waste management (pp. 133–151). Berlin: Springer. https://doi.org/10.1007/978-3-319-57076-1
Eiselt, H. A., & Marianov, V. (2014). A bi-objective model for the location of landfills for municipal solid waste. European Journal of Operational Research, 235, 187–194. https://doi.org/10.1016/j.ejor.2013.10.005
Eiselt, H. A., & Marianov, V. (2015). Location modeling for municipal solid waste facilities. Computers and Operations Research, 62, 305–315. https://doi.org/10.1016/j.cor.2014.05.003
Erazo, J., Villaroel, H. (2015). Ubicación y determinación de los parámetros de diseño de las estaciones de transferencia de residuos sólidos para la mancomunidad Mundo Verde en las provincias de Guayas, Los Ríos y Bolívar. Universidad Central del Ecuador. Doi: https://doi.org/10.1145/3132847.3132886
Farahani, R. Z., Fallah, S., Ruiz, R., Hosseini, S., & Asgari, N. (2019). OR models in urban service facility location: A critical review of applications and future developments. European Journal of Operational Research, 276, 1–27. https://doi.org/10.1016/j.ejor.2018.07.036
Feizizadeh, B., & Blaschke, T. (2014). An uncertainty and sensitivity analysis approach for GIS-based multicriteria landslide susceptibility mapping. International Journal of Geographical Information Science, 28(3), 610–638. https://doi.org/10.1080/13658816.2013.869821
Feizizadeh, B., Ronagh, Z., Pourmoradian, S., Gheshlaghi, H. A., Lakes, T., & Blaschke, T. (2021). An efficient GIS-based approach for sustainability assessment of urban drinking water consumption patterns: A study in Tabriz city. Iran. Sustainable Cities and Society, 64, 102584. https://doi.org/10.1016/j.scs.2020.102584
Ferronato, N., Rada, E. C., Gorritty Portillo, M. A., Cioca, L. I., Ragazzi, M., & Torretta, V. (2019). Introduction of the circular economy within developing regions: A comparative analysis of advantages and opportunities for waste valorization. Journal of Environmental Management, 230, 366–378. https://doi.org/10.1016/j.jenvman.2018.09.095
Galan, B., Dosal, E., Andrés, A., & Viguri, J. (2013). Optimisation of the construction and demolition waste management facilities location in Cantabria (Spain) under economical and environmental criteria. Waste and Biomass Valorization, 4, 797–808. https://doi.org/10.1007/s12649-013-9196-0
Galante, G., Aiello, G., Enea, M., & Panascia, E. (2010). A multi-objective approach to solid waste management. Waste Management, 30, 1720–1728. https://doi.org/10.1016/j.wasman.2010.01.039
García, M. (2008). Plantas o estaciones de transferencia (p. 22). https://static.eoi.es/savia/documents/componente45914.pdf.
Ghajari, Y. E., Alesheikh, A. A., Modiri, M., Hosnavi, R., & Abbasi, M. (2017). Spatial modelling of urban physical vulnerability to explosion hazards using GIS and fuzzy MCDA. Sustainability (Switzerland), 9(7), 1–29. https://doi.org/10.3390/su9071274
Ghiani, G., Laganà, D., Manni, E., Musmanno, R., & Vigo, D. (2014). Operations research in solid waste management: A survey of strategic and tactical issues. Computers and Operations Research, 44, 22–32. https://doi.org/10.1016/j.cor.2013.10.006
Gil, Y., & Kellerman, A. (1993). A multicriteria model for the location of solid waste transfer stations : The case of ashdod Israel. GeoJournal, 29, 377–384. https://doi.org/10.1007/BF00807540
González, G. (2010). Tratamiento y disposicion final de residuos sólidos en Argentina. Tratamiento y disposición final. Situación actual y alternativos futuros.
Gough, M. Z., & Accordino, J. (2013). Public gardens as sustainable community development partners: Motivations, perceived benefits, and challenges. Urban Affairs Review, 49, 851–887. https://doi.org/10.1177/1078087413477634
Greco, G., Allegrini, M., Del Lungo, C., Gori Savellini, P., & Gabellini, L. (2015). Drivers of solid waste collection costs. Empirical evidence from Italy. Journal of Cleaner Production, 106, 364–371. https://doi.org/10.1016/j.jclepro.2014.07.011
Habibi, F., Asadi, E., Sadjadi, S. J., & Barzinpour, F. (2017). A multi-objective robust optimization model for site-selection and capacity allocation of municipal solid waste facilities: A case study in Tehran. Journal of Cleaner Production, 166, 816–834. https://doi.org/10.1016/j.jclepro.2017.08.063
Hariz, H. A., Sennaroglu, B., & Sennaroglu, B. (2017). Siting of a central healthcare waste incinerator using GIS-Based Multi- Criteria decision analysis. Journal of Cleaner Production, 166, 1031–1042. https://doi.org/10.1016/j.jclepro.2017.08.091
Hasbiah, A. W., Nugroho, F. L., Juliana, J. (2021). Bacteriological air quality at transfer station area (case study: Gegerkalong Transfer Station, Bandung City). IOP Conference Series: Earth and Environmental Science. Doi: https://doi.org/10.1088/1755-1315/737/1/012066
Hernández, M., Rodríguez, J. (2016). Identificación de áreas potenciales para el emplazamiento de estaciones de transferencia de residuos sólidos ordinarios para la zona urbana de la ciudad de Bogatá D.C, por medio del análsis y correlación de variables influyentes, en un sistema de informac. Universidad de la Salle.
Hettiarachchi, H., Ryu, S., Caucci, S., & Silva, R. (2018). Municipal solid waste management in Latin America and the Caribbean: Issues and potential solutions from the governance perspective. Recycling. https://doi.org/10.3390/recycling3020019
Höke, M. C., & Yalcinkaya, S. (2021). Municipal solid waste transfer station planning through vehicle routing problem-based scenario analysis. Waste Management and Research, 39, 185–196. https://doi.org/10.1177/0734242X20966643
Huang, I. B., Keisler, J., & Linkov, I. (2011). Multi-criteria decision analysis in environmental sciences: Ten years of applications and trends. Science of the Total Environment, 409, 3578–3594. https://doi.org/10.1016/j.scitotenv.2011.06.022
Hussain, F., Nawaz, M., & Adila, S. (2014). Assessment of key parameters in municipal solid waste management: A prerequisite for sustainability. International Journal of Sustainable Development and World Ecology, 21, 519–525. https://doi.org/10.1080/13504509.2014.971452
Hyde, K., Maier, H. R., & Colby, C. (2003). Incorporating uncertainty in the PROMETHEE MCDA method. Journal of Multi-Criteria Decision Analysis, 12(4–5), 245–259. https://doi.org/10.1002/mcda.361
Jaiswal, A., & Bharat, A. (2016). Exploring criteria to locate solid waste Transfer station in an urban area. Journal of Solid Waste Technology and Management, 42, 58–65. https://doi.org/10.5276/JSWTM.2016.58
Josimović, B., Marić, I., & Milijić, S. (2015). Multi-criteria evaluation in strategic environmental assessment for waste management plan, a case study: The city of Belgrade. Waste Management, 36, 331–342. https://doi.org/10.1016/j.wasman.2014.11.003
Kaghembega, S. W., Xia, Q., Chen, S., & Chaofei, S. (2020). Greenhouse gas reduction and cost-benefit through improving municipal solid waste management in Ouagadougou. African Journal of Environmental Science and Technology, 14, 222–230. https://doi.org/10.5897/AJEST2020.2878
Kahraman, C., Cebi, S., Onar, S. C., & Oztaysi, B. (2018). A novel trapezoidal intuitionistic fuzzy information axiom approach: An application to multicriteria landfill site selection. Engineering Applications of Artificial Intelligence, 67, 157–172. https://doi.org/10.1016/j.engappai.2017.09.009
Kamdar, I., Ali, S., Bennui, A., Techato, K., & Jutidamrongphan, W. (2019). Municipal solid waste landfill siting using an integrated GIS-AHP approach: A case study from Songkhla, Thailand. Resources, Conservation and Recycling, 149, 220–235. https://doi.org/10.1016/j.resconrec.2019.05.027
Kannangara, M., Dua, R., Ahmadi, L., & Bensebaa, F. (2018). Modeling and prediction of regional municipal solid waste generation and diversion in Canada using machine learning approaches. Waste Management, 74, 3–15. https://doi.org/10.1016/j.wasman.2017.11.057
Karim Ghani, W. A. W. A., Rusli, I. F., Biak, D. R. A., & Idris, A. (2013). An application of the theory of planned behaviour to study the influencing factors of participation in source separation of food waste. Waste Management, 33, 1276–1281. https://doi.org/10.1016/j.wasman.2012.09.019
Khadivi, M. R., & Ghomi, S. M. T. F. (2012). Solid waste facilities location using of analytical network process and data envelopment analysis approaches. Waste Management, 32, 1258–1265. https://doi.org/10.1016/j.wasman.2012.02.002
Khan, M., Vaezi, M., & Kumar, A. (2018). Optimal siting of solid waste-to-value-added facilities through a GIS-based assessment. Science of the Total Environment, 610–611, 1065–1075. https://doi.org/10.1016/j.scitotenv.2017.08.169
Khoshbeen, A. R., Logan, M., & Visvanathan, C. (2020). Integrated solid-waste management for Kabul city, Afghanistan. Journal of Material Cycles and Waste Management, 22, 240–253. https://doi.org/10.1007/s10163-019-00936-z
Kirca, O., & Erkip, N. (1988). Case Study Selecting transfer station locations for large solid waste systems. European Journal of Operational Research, 35, 339–349. https://doi.org/10.1016/0377-2217(88)90224-X
Kůdela, J., Šomplák, R., Nevrlý, V., Lipovský, T., Smejkalová, V., & Dobrovský, L. (2019). Multi-objective strategic waste transfer station planning. Journal of Cleaner Production, 230, 1294–1304. https://doi.org/10.1016/j.jclepro.2019.05.167
Kumar, A., Wasan, P., Luthra, S., & Dixit, G. (2020). Development of a framework for selecting a sustainable location of waste electrical and electronic equipment recycling plant in emerging economies. Journal of Cleaner Production, 277, 122645. https://doi.org/10.1016/j.jclepro.2020.122645
Kumler, M., Goodchild, M. (1992). The population center of Canada - just north of Toronto. Geographical Snapshots of North America 275–279.
Lella, J., Mandla, V. R., & Zhu, X. (2017). Solid waste collection/transport optimization and vegetation land cover estimation using Geographic Information System (GIS): A case study of a proposed smart-city. Sustainable Cities and Society, 35, 336–349. https://doi.org/10.1016/j.scs.2017.08.023
Leonelli, R. B. (2012). Enhancing a decision support tool with sensitivit Y analysis. University of Manchester.https://studentnet.cs.manchester.ac.uk/resources/library/thesis_abstracts/MSc12/FullText/BertuzziLeonelli-RenzoCristian-fulltext.pdf
Li, Y., & Huang, G. (2010). Modeling municipal solid waste management system under uncertainty. Journal of the Air and Waste Management Association, 60, 439–453. https://doi.org/10.3155/1047-3289.60.4.439
Li, Y. P., Huang, G. H., Cui, L., & Liu, J. (2019). Mathematical modeling for identifying cost-effective policy of municipal solid waste management under uncertainty. Journal of Environmental Informatics, 34, 55–67. https://doi.org/10.3808/jei.201900417
Lin, Z., Xie, Q., Feng, Y., Zhang, P., & Yao, P. (2020). Towards a robust facility location model for construction and demolition waste transfer stations under uncertain environment: The case of Chongqing. Waste Management, 105, 73–83. https://doi.org/10.1016/j.wasman.2020.01.037
Liu, J., & Zheng, G. (2020). Emission of volatile organic compounds from a small-scale municipal solid waste transfer station: Ozone-formation potential and health risk assessment. Waste Management, 106, 193–202. https://doi.org/10.1016/j.wasman.2020.03.031
Liu, K., Lin, S., Hsieh, J., & Tzeng, G. (2018). Improving the food waste composting facilities site selection for sustainable development using a hybrid modified MADM model. Waste Management, 75, 44–59. https://doi.org/10.1016/j.wasman.2018.02.017
Louati, A., Son, L. H., & Chabchoub, H. (2019). Smart routing for municipal solid waste collection: A heuristic approach. Journal of Ambient Intelligence and Humanized Computing, 10, 1865–1884. https://doi.org/10.1007/s12652-018-0778-3
Municipal Public Cleaning Company of Cuenca (EMAC-EP), 2006. Documentos técnicos para la implementación de una estación de transferencia de residuos para la ciudad de Cuenca. Empresa Municipal de Aseo de Cuenca (EMAC-EP).
Madruga de Brito, M., Almoradie, A., & Evers, M. (2019). Spatially-explicit sensitivity and uncertainty analysis in a MCDA-based flood vulnerability model. International Journal of Geographical Information Science, 33(9), 1788–1806. https://doi.org/10.1080/13658816.2019.1599125
Makarichi, L., Techato, K., & Jutidamrongphan, W. (2018). Material flow analysis as a support tool for multi-criteria analysis in solid waste management decision-making. Resources, Conservation and Recycling, 139, 351–365. https://doi.org/10.1016/j.resconrec.2018.07.024
Maliene, V., Dixon-Gough, R., & Malys, N. (2018). Dispersion of relative importance values contributes to the ranking uncertainty: Sensitivity analysis of multiple criteria decision-making methods. Applied Soft Computing Journal, 67, 286–298. https://doi.org/10.1016/j.asoc.2018.03.003
Mamashli, Z., & Javadian, N. (2021). Sustainable design modifications municipal solid waste management network and better optimization for risk reduction analyses. Journal of Cleaner Production, 279, 123824. https://doi.org/10.1016/j.jclepro.2020.123824
Mantzaras, G., & Voudrias, E. A. (2017). An optimization model for collection, haul, transfer, treatment and disposal of infectious medical waste: Application to a Greek region. Waste Management, 69, 518–534. https://doi.org/10.1016/j.wasman.2017.08.037
Marshall, R. E., & Farahbakhsh, K. (2013). Systems approaches to integrated solid waste management in developing countries. Waste Management, 33, 988–1003. https://doi.org/10.1016/j.wasman.2012.12.023
Marttunen, M., Lienert, J., & Belton, V. (2017). Structuring problems for multi-criteria decision analysis in practice: A literature review of method combinations. European Journal of Operational Research, 263, 1–17. https://doi.org/10.1016/j.ejor.2017.04.041
Massukado, L. M. (2004). Sistema de Apoio à Decisão: avaliação de cenários de gestão integrada de resíduos sólidos urbanos domiciliares. Universidad Federal de Sao Carlos
Medina, A. (2009). Diseño de estaciones de transferencia de residuos sólidos para los municipios de Atoyac de Álvarez, Benito Juárez y Tecpan de Galeana. Universidad NAcional Autónoma de Mexico.
Merchán, A., Pomavilla, M. (2019). Diagnóstico ambiental de disposición final de desechos sólidos comunes y sanitarios de Camilo Ponce Enríquez y diseño del relleno sanitario. Universidad Técnica de Machala.
Ministry of Human Development of Bolivia. (1996). Norma Boliviana NB 742–760: Normas de residuos sólidos. IBNORCA, Bolivia.
Ministry of Environment and Natural Resources of Mexico. (2010). Criterios para la ubicación, operación y cierre de infraestructura ambiental para el acopio, transferencia, separación y tratamiento de residuos sólidos urbanos y de manejo especial. https://www.gob.mx/semarnat/acciones-y-programas/comunicacion-y-difusion
Ministry of the Environment of Ecuador. (2010). Norma de calidad ambiental para el manejo y disposición final de desechos sólidos no peligrosos.
Ministry of the Environment of Ecuador. (2017). Código Orgánico del Ambiente.
Ministry of the Environment of Peru. (2017). Reglamento del decreto legislativo N°1278: Decreto legislativo que aprueba la ley de gestión integral de residuos sólidos.
Moeinaddini, M., Khorasani, N., Danehkar, A., Darvishsefat, A. A., & Zienalyan, M. (2010). Siting MSW landfill using weighted linear combination and analytical hierarchy process (AHP) methodology in GIS environment (case study: Karaj). Waste Management, 30, 912–920. https://doi.org/10.1016/j.wasman.2010.01.015
Mofid-Nakhaee, E., Barzinpour, F., & Pishvaee, M. S. (2020). A sustainable municipal solid waste system design considering public awareness and education: A case study. Waste Management and Research, 38, 626–638. https://doi.org/10.1177/0734242X20910206
Mohamadzadeh, P., Pourmoradian, S., Feizizadeh, B., Sharifi, A., & Vogdrup-Schmidt, M. (2020). A gis-based approach for spatially-explicit sustainable development assessments in East Azerbaijan Province Iran. Sustainability (switzerland), 12(24), 1–16. https://doi.org/10.3390/su122410413
Mohsenizadeh, M., Tural, M. K., & Kentel, E. (2020). Municipal solid waste management with cost minimization and emission control objectives: A case study of Ankara. Sustainable Cities and Society, 52, 101807. https://doi.org/10.1016/j.scs.2019.101807
Monzambe, G. M., Mpofu, K., & Daniyan, I. A. (2021). Optimal location of landfills and transfer stations for municipal solid waste in developing countries using non-linear programming. Sustainable Futures, 3, 100046. https://doi.org/10.1016/j.sftr.2021.100046
National Assembly of the Republic of Ecuador, 2010. Código Orgánico Organización Territorial Autonomía Descentralización COOTAD. In Registro Oficial Suplemento 303 de 19-oct-2010.
National Institute of Statistics and Censuses of Ecuador, 2012. Población y Demografía. Población y Tasas de Crecimiento Intercensal de 2010–2001–1990 Por Sexo, Según Parroquias.
National Institute of Preinvestment of Ecuador. (2013). Términos de referencia de estudios de prefactibilidad, factibilidad y diseños definitivos para la gestión integral y aprovechamiento de los desechos sólidos generados por la Mancomunidad Mundo Verde o del Buen Vivir O Sumak Kawsay. Quito.
National Institute of Statistics and Censuses of Ecuador, 2020. Estadística Ambiental Económica en Gobiernos Autónomos Descentralizados Municipales. Gestión de Residuos Sólidos 2019. Gestión de Residuos Sólidos, 26.
Oktrianto, D., & Siswanto, N. (2017). Determination of provincial level of hazardous waste collection location in East Java province using center of gravity method. International Journal of Innovative Science and Research Technology, 2, 304–309. https://doi.org/10.22515/sustinere.jes.v1i2.16
Ortega, Y., Torres, S. (2016). Diseño de una planta de tratamiento de residuos sólidos municipales para poblaciones pequeñas. Universidad Nacional del Centro del Perú.
Özkan, B., Sarıçiçek, İ, & Özceylan, E. (2020). Evaluation of landfill sites using GIS-based MCDA with hesitant fuzzy linguistic term sets. Environmental Science and Pollution Research, 27, 42908–42932. https://doi.org/10.1007/s11356-020-10128-0
Pan, A., Yu, L., & Yang, Q. (2019). Characteristics and forecasting of municipal solid waste generation in China. Sustainability. https://doi.org/10.3390/su11051433
Paul, K., Chattopadhyay, S., Dutta, A., Krishna, A. P., & Ray, S. (2020). Developing and optimising an urban integrated solid waste management model: Effect of transfer stations. Journal of Urban and Environmental Engineering, 14, 119–131. https://doi.org/10.4090/juee.2020.v14n1.119131
Perdomo, J., Ramirez, J. (2011). Análisis económico sobre el tamaño óptimo del mercado y ubicación de estaciones de transferencia para el manejo de residuos sólidos en Colombia. Munich Personal RePEc Archive (MPRA) 143–162.
Pluskal, J., Šomplák, R., Nevrlý, V., Smejkalová, V., & Pavlas, M. (2020). Strategic decisions leading to sustainable waste management: Separation, sorting and recycling possibilities. Journal of Cleaner Production, 278, 1–16. https://doi.org/10.1016/j.jclepro.2020.123359
Presidency of the Republic of El Salvador, 2002. Reglamento especial sobre el manejo integral de los desechos sólidos y sus anexos. 13.
Presidency of the Republic of Nicaragua, 2002. Norma técnica para el control ambiental de los rellenos sanitarios para desechos sólidos no peligrosos.
Presidency of the Republic of Costa Rica, 2014. Decreto Ejecutivo N° 38928-S: Reglamento sobre rellenos sanitarios. http://extwprlegs1.fao.org/docs/pdf/cos148805.pdf
Rafiee, R., Horasani, N., Mahiny, A., Darvishsefat, A., Danekar, A., & Hasad, S. (2011). Siting transfer stations for municipal solid waste using a spatial multi-criteria analysis. Environmental and Engineering Geoscience, 17, 143–154. https://doi.org/10.2113/gseegeosci.17.2.143
Rahimi, S., Hafezalkotob, A., Monavari, S. M., Hafezalkotob, A., & Rahimi, R. (2020). Sustainable landfill site selection for municipal solid waste based on a hybrid decision-making approach: Fuzzy group BWM-MULTIMOORA-GIS. Journal of Cleaner Production, 248, 119186. https://doi.org/10.1016/j.jclepro.2019.119186
Randazzo, L., Cusumano, A., Oliveri, G., Di Stefano, P., Renda, P., Perricone, M., & Zarcone, G. (2018). Landfill site selection for municipal solid waste by using AHP method in GIS environment: Waste management decision-support in sicily (Italy). Detritus, 2, 78–88. https://doi.org/10.31025/2611-4135/2018.13656
Rathore, P., & Sarmah, S. (2019). Modeling transfer station locations considering source separation of solid waste in urban centers: A case study of Bilaspur city, India. Journal of Cleaner Production, 211, 44–60. https://doi.org/10.1016/j.jclepro.2018.11.100
Ministry of the Environment of Guatemala, 2016. Reglamento para el manejo integral de residuos sólidos. Guatemala.
Ribas, G. (2019). Diseño de una planta de tratamiento de residuos sólidos urbanos para la ciudad de Gálvez. Universidad Tecnológica Nacional.
Roé, A., Rojas, M., & Torres, C. (2014). Localización De Un Sitio Para Construir Un Centro De Aprovechamiento De Residuos Sólidos Urbanos a Través De Tres Métodos. Revista AIDIS de Ingeniería y Ciencias Ambientales: Investigación, Desarrollo y Práctica, 7, 141–153. https://doi.org/10.22201/iingen.0718378xe.2014.7.2.46796
Saaty, T. L. (1994). Fundamentals of Decision Making and Priority Theory With the Analytic Hierarchy Process, Vol. 6.
Saaty, T. L. (1980). The analytic hierarchy process: Planning, priority setting. Resource Allocation. McGraw-Hill International Book Company.
Saavedra, O., Nogales, O. (1998). Nuevo emplazamiento del relleno sanitario para el municipio de Cochabamba, in: XXXII Congreso Interamericano de Ingenieria Sanitaria y Ambiental-At: Lima, Peru. Cochabamba, p. 13.
Sahir, S., Rosmawati, R., & Minan, K. (2017). Simple additive weighting method to determining employee salary increase rate. International Journal of Scientific Research in Science and Technology (IJSRST), 3, 42–48. https://doi.org/10.32628/IJSRST1173821
Sánchez, J., Estrada, R. (1996). Estaciones de transferencia de residuos sólidos en áreas urbanas. In Instituto Nacional de Ecología. México.
Santos, R., De, C., Rodrigues, S., Pereira, A. B., Rabelo, J. M., Reis, R. L., Santos, D., De Souza, R. C., Marcos, J., & Rabelo, G. (2021). Multicriteria Decision Analysis (MCDA) and Geographic Information System (GIS) in Construction and Demolition Waste (CDW) Management. International Journal of Science and Engineering Investigations, 10, 43–48.
Sarkhosh, M., Shamsipour, A., Yaghmaeian, K., Nabizadeh, R., Naddafi, K., & Mohseni, S. M. (2017). Dispersion modeling and health risk assessment of VOCs emissions from municipal solid waste transfer station in Tehran Iran. Journal of Environmental Health Science and Engineering, 15, 1–7. https://doi.org/10.1186/s40201-017-0268-0
Secretariat of Social Development of Mexico, 2001. Manual técnico sobre generación, recolección y transferencia de residuos sólidos municipales.
Sellak, H., Ouhbi, B., & Frikh, B. (2018). A knowledge-based outranking approach for multi-criteria decision-making with hesitant fuzzy linguistic term sets. Applied Soft Computing Journal, 67, 625–640. https://doi.org/10.1016/j.asoc.2017.06.031
Shi, Q., Ren, H., Ma, X., & Xiao, Y. (2019). Site selection of construction waste recycling plant. Journal of Cleaner Production, 227, 532–542. https://doi.org/10.1016/j.jclepro.2019.04.252
Shyamali, G., & Kumar, R. S. (2021). Fuzzy-Rough multi-objective product blending fixed-charged transportation problem with truck load constraints through transfer station. RAIRO Operations Research. https://doi.org/10.1051/ro/2020129
Siclari, P., Olivotto, V. (2021). Gestión local del cambio climático: planificación participativa y gobernanza territorial. FLACSO Ecuador, Quito - Ecuador.
Singh, A. (2019). Remote sensing and GIS applications for municipal waste management. Journal of Environmental Management, 243, 22–29. https://doi.org/10.1016/j.jenvman.2019.05.017
Singh, A., & Vidyarthi, A. K. (2008). Optimal allocation of landfill disposal site: A fuzzy multi-criteria approach. Journal of Environmental Health Science & Engineering, 5, 25–34.
Solangi, Y. A., Tan, Q., Khan, M. W. A., Mirjat, N. H., & Ahmed, I. (2018). The selection of wind power project location in the Southeastern Corridor of Pakistan: A factor analysis, AHP, and fuzzy-TOPSIS application. Energies. https://doi.org/10.3390/en11081940
Solíz, T. M. F. (2015). Ecología política y geografía crítica de la basura en el Ecuador Letras Verdes. Revista Latinoamericana de Estudios Socioambientales, 17, 4–28. https://doi.org/10.17141/letrasverdes.17.2015.1259
Son, L. H., & Louati, A. (2016). Modeling municipal solid waste collection: A generalized vehicle routing model with multiple transfer stations, gather sites and inhomogeneous vehicles in time windows. Waste Management, 52, 34–49. https://doi.org/10.1016/j.wasman.2016.03.041
Sultan, M., Waheed, S., Ali, U., Sweetman, A. J., Jones, K. C., & Malik, R. N. (2019). Insight into occurrence, profile and spatial distribution of organochlorine pesticides in soils of solid waste dumping sites of Pakistan: Influence of soil properties and implications for environmental fate. Ecotoxicology and Environmental Safety, 170, 195–204. https://doi.org/10.1016/j.ecoenv.2018.11.020
Sun, Z., Cheng, Z., Wang, L., Lou, Z., Zhu, N., Zhou, X., & Feng, L. (2017). The typical MSW odorants identification and the spatial odorants distribution in a large-scale transfer station. Environmental Science and Pollution Research, 24, 7705–7713. https://doi.org/10.1007/s11356-017-8455-1
Tan, Q., Huang, G. H., & Cai, Y. P. (2010). Identification of optimal plans for municipal solid waste management in an environment of fuzziness and two-layer randomness. Stochastic Environmental Research and Risk Assessment, 24, 147–164. https://doi.org/10.1007/s00477-009-0307-1
Tan, Q., Huang, G. H., & Cai, Y. P. (2010). Waste management with recourse: An inexact dynamic programming model containing fuzzy boundary intervals in objectives and constraints. Journal of Environmental Management, 91, 1898–1913. https://doi.org/10.1016/j.jenvman.2010.04.005
Tapia, J. (2008). Metodología para el diseño de una estación de transferencia de residuos sólidos en áreas urbanas. Universidad Internacional SEK
Tavares, G., Zsigraiová, Z., & Semiao, V. (2011). Multi-criteria GIS-based siting of an incineration plant for municipal solid waste. Waste Management, 31, 1960–1972. https://doi.org/10.1016/j.wasman.2011.04.013
Tello, P., Martínez, E., Daza, D., Soulier, M,, Terraza, H. (2010). Informe de la evaluación regional del manejo de residuos sólidos urbanos en América Latina y el Caribe 2010.
Thampi, A., & Rao, B. (2015). Application of multi-criteria decision making tools for technology choice in treatment and disposal of municipal solid waste for local self government bodies - A case study of Kerala, India. Journal of Solid Waste Technology and Management, 41, 84–95. https://doi.org/10.5276/JSWTM.2015.84
Turcott Cervantes, D. E., López Martínez, A., Cuartas Hernández, M., García, L., & de Cortázar, A. (2018). Using indicators as a tool to evaluate municipal solid waste management: A critical review. Waste Management, 80, 51–63. https://doi.org/10.1016/j.wasman.2018.08.046
United Nations General Assembly, 2005. 60/1. 2005 World Summit Outcome.
Urriago, N. (2018). Estudio de pre-factibilidad de implementación de una estación de transferencia con recuperación de residuos sólidos urbanos en la UPZ-39 Quiroga, localidad 18 Rafael Uribe Uribe de la ciudad de Bogotá. Universidad Nacional Abierta y a Distancia.
Varón, K., Orejuela, J., & Manyoma, P. (2015). Modelo matemático para la ubicación de estaciones de transferencia de residuos sólidos urbanos. Revista EIA, 12, 61–70. https://doi.org/10.24050/reia.v12i23.721
Vásquez, O. (2005). Modelo de simulación de gestión de residuos sólidos domiciliarios en la Región Metropolitana de Chile. Revista De Dinámica De Sistemas, 1, 27–52.
Vega, K. (2016). Mancomunidad alternativa financiera y económica válida para la Gestión Integral de Residuos Sólidos Casos Cantones San Pedro de Pelileo y Patate. Pontificia Universidad Católica del Ecuador.
Veitia, E., Montalvan, A., & Martínez, Y. (2014). Elección de indicadores sistémicos para la sostenibilidad ambiental del suelo. Revista Ciencias Técnicas Agropecuarias, 23, 43–50.
Vitorino, A., Montenegro, S., Faceli, K., & Casadei, V. (2017). Technologies and decision support systems to aid solid-waste management: A systematic review. Waste Management, 59, 567–584. https://doi.org/10.1016/j.wasman.2016.10.045
Vucijak, B., Kurtagic, S., & Silajdzic, I. (2015). Multicriteria decision making in selecting best solid waste management scenario : A municipal case study from Bosnia and Herzegovina. Journal of Cleaner Production, 130, 166–174. https://doi.org/10.1016/j.jclepro.2015.11.030
Waste Agency of Catalan, 2016. Guía práctica para el diseño y la explotación de plantas de compostaje.
Wang, H., Zhang, B., Liu, Y., Liu, Y., Xu, S., Zhao, Y., Chen, Y., & Hong, S. (2020). Urban expansion patterns and their driving forces based on the center of gravity-GTWR model: A case study of the Beijing-Tianjin-Hebei urban agglomeration. Journal of Geographical Sciences, 30, 297–318. https://doi.org/10.1007/s11442-020-1729-4
Wang, K., Zhao, X., Peng, B., & Zeng, Y. (2020). Spatio-temporal pattern and driving factors of municipal solid waste generation in China: New evidence from exploratory spatial data analysis and dynamic spatial models. Journal of Cleaner Production, 270, 121794. https://doi.org/10.1016/j.jclepro.2020.121794
World Bank. (2018). what a waste 2.0 a global sanpshot of solid waste management to 2050. Washington DC. https://doi.org/10.1596/978-1-4648-1329-0
World Commission on Environment and Development (WCED). (1987). Sustainable Development: A Guide to Our Common Future: the Report of the World Commission on Environment and Development (Issue August). https://digitallibrary.un.org/record/139811
Wu, Y., Chen, K., Zeng, B., Yang, M., & Geng, S. (2016). Cloud-based decision framework for waste-to-energy plant site selection – A case study from China. Waste Management, 48, 593–603. https://doi.org/10.1016/j.wasman.2015.11.030
Yadav, V., Bhurjee, A. K., Karmakar, S., & Dikshit, A. K. (2017). A facility location model for municipal solid waste management system under uncertain environment. Science of the Total Environment, 603–604, 760–771. https://doi.org/10.1016/j.scitotenv.2017.02.207
Yadav, V., Kalbar, P. P., Karmakar, S., & Dikshit, A. K. (2020). A two-stage multi-attribute decision-making model for selecting appropriate locations of waste transfer stations in urban centers. Waste Management, 114, 80–88. https://doi.org/10.1016/j.wasman.2020.05.024
Yadav, V., & Karmakar, S. (2020). Sustainable collection and transportation of municipal solid waste in urban centers. Sustainable Cities and Society, 53, 101937. https://doi.org/10.1016/j.scs.2019.101937
Yadav, V., Karmakar, S., Dikshit, A. K., & Bhurjee, A. K. (2018). Interval-valued facility location model: An appraisal of municipal solid waste management system. Journal of Cleaner Production, 171, 250–263. https://doi.org/10.1016/j.jclepro.2017.09.233
Yadav, V., Karmakar, S., Dikshit, A. K., & Vanjari, S. (2016). A feasibility study for the locations of waste transfer stations in urban centers: A case study on the city of Nashik, India. Journal of Cleaner Production, 126, 191–205. https://doi.org/10.1016/j.jclepro.2016.03.017
Yalcinkaya, S. (2020). A spatial modeling approach for siting, sizing and economic assessment of centralized biogas plants in organic waste management. Journal of Cleaner Production, 255, 120040. https://doi.org/10.1016/j.jclepro.2020.120040
Yalcinkaya, S., & Kirtiloglu, O. S. (2021). Application of a geographic information system-based fuzzy analytic hierarchy process model to locate potential municipal solid waste incineration plant sites: A case study of Izmir Metropolitan Municipality. Waste Management and Research, 39, 174–184. https://doi.org/10.1177/0734242X20939636
Yalew, S. G., van Griensven, A., & van der Zaag, P. (2016). AgriSuit: A web-based GIS-MCDA framework for agricultural land suitability assessment. Computers and Electronics in Agriculture, 128, 1–8. https://doi.org/10.1016/j.compag.2016.08.008
Yong, T., Tung, L., Tan, K. (2009). Integrated solid waste management. In Clean, green and blue: Singapore´s Journey Towards Environmental and Water Sustainability. ISEAS, Singapore.
Yu, H., & Solvang, W. D. (2017). A multi-objective location-allocation optimization for sustainable management of municipal solid waste. Environment Systems and Decisions, 37, 289–308. https://doi.org/10.1007/s10669-017-9632-y
Zanghelini, G., Cherubini, E., & Soares, S. (2018). How Multi-Criteria Decision Analysis (MCDA) is aiding Life Cycle Assessment (LCA) in results interpretation. Journal of Cleaner Production, 172, 609–622. https://doi.org/10.1016/j.jclepro.2017.10.230
Zarin, R., Azmat, M., Naqvi, S. R., Saddique, Q., & Ullah, S. (2021). Landfill site selection by integrating fuzzy logic, AHP, and WLC method based on multi-criteria decision analysis. Environmental Science and Pollution Research, 28, 19726–19741. https://doi.org/10.1007/s11356-020-11975-7
Zhang, L., Chen, J., Hao, Q., & Li, C. Z. (2018). Measuring the NIMBY effect in urban China: The case of waste transfer stations in metropolis Shanghai. Journal of Housing and the Built Environment. https://doi.org/10.1007/s10901-017-9565-2
Zhang, Y., Zhang, J., Yang, Z., & Li, J. (2012). Analysis of the distribution and evolution of energy supply and demand centers of gravity in China. Energy Policy, 49, 695–706. https://doi.org/10.1016/j.enpol.2012.07.012
Acknowledgements
The authors want to thank Universidad Católica de Cuenca and Municipal Public Cleaning Company of Cuenca (EMAC-EP) for their financial support. Besides, our acknowledgment goes to José Solano-Peláez for his support during the research process; Tania Bustamante for the English editing service; Computational Calculation, Modeling and Data Analytics (C2MAD) and DatAchademics laboratories.
Funding
This work was supported by Universidad Católica de Cuenca and Municipal Public Cleaning Company of Cuenca (EMAC-EP) through the project “Joint system of transportation, treatment and final disposal of solid waste for the province of Azuay”.
Author information
Authors and Affiliations
Contributions
Sandra L. Cobos-Mora involved in conceptualization, methodology, formal analysis, investigation, writing—original draft, supervision, funding acquisition, and project administration José Guamán-Aucapiña involved in methodology, software, validation, formal analysis, investigation, resources, data curation, and writing—original Draft Jonathan Zúñiga-Ruiz involved in methodology, validation, formal analysis, investigation, and resources.
Corresponding author
Ethics declarations
Conflict of interest
Not applicable.
Ethical approval
Not applicable.
Consent to participate
Not applicable.
Consent for publication
Not applicable.
Additional information
Publisher's Note
Springer Nature remains neutral with regard to jurisdictional claims in published maps and institutional affiliations.
The article has been updated: Retrospective open access has been processed for the article.
Appendix
Appendix
Abbreviation | Description |
---|---|
AHP | Analytic Hierarchy Process |
FDSs | Final Disposal Sites |
GC | Gravity Center |
GIS | Geographical Information Systems |
GIS-MCDA | Multi-criteria Decision Analysis based on Geographical Information Systems |
ISWM | Integrated Solid Waste Management |
MCDA | Multi-criteria Decision Analysis |
MILP | Mixed-integer linear programming |
MINLP | Mixed-integer nonlinear programming |
MOMIP | Multi-Objective Mixed-Integer Programming |
MSW | Municipal Solid Waste |
MSWM | Municipal Solid Waste Management |
NIMBY | Not-in-my-backyard |
TSI | Territory Suitability Index |
TSs | Transfer Stations |
Rights and permissions
Open Access This article is licensed under a Creative Commons Attribution 4.0 International License, which permits use, sharing, adaptation, distribution and reproduction in any medium or format, as long as you give appropriate credit to the original author(s) and the source, provide a link to the Creative Commons licence, and indicate if changes were made. The images or other third party material in this article are included in the article's Creative Commons licence, unless indicated otherwise in a credit line to the material. If material is not included in the article's Creative Commons licence and your intended use is not permitted by statutory regulation or exceeds the permitted use, you will need to obtain permission directly from the copyright holder. To view a copy of this licence, visit http://creativecommons.org/licenses/by/4.0/.
About this article
Cite this article
Cobos-Mora, S.L., Guamán-Aucapiña, J. & Zúñiga-Ruiz, J. Suitable site selection for transfer stations in a solid waste management system using analytical hierarchy process as a multi-criteria decision analysis: a case study in Azuay-Ecuador. Environ Dev Sustain 25, 1944–1977 (2023). https://doi.org/10.1007/s10668-022-02134-8
Received:
Accepted:
Published:
Issue Date:
DOI: https://doi.org/10.1007/s10668-022-02134-8