Abstract
Climate change and the ongoing energy transition can increase energy poverty rates. To date, the main tool employed to alleviate energy poverty has involved income transfers to vulnerable households. However, measures that seek to improve a home’s energy efficiency have recently gained increasing relevance. In this study we assess the effectiveness of these two types of policy, assuming universal coverage and optimal behaviour. Results points that income transfers and energy efficiency measures have the potential to decrease the proportion of households in energy poverty; however, the magnitude of their respective effects differs greatly. The average impact of energy efficiency measures provides for a greater reduction in energy poverty rates than income transfer policies. Although the greatest reduction in energy poverty is obtained by combining both measures, this combination of tools leads to overlapping effects with income transfers making only a marginal contribution once total retrofit have been implemented.
Similar content being viewed by others
Avoid common mistakes on your manuscript.
1 Introduction
Although guaranteeing access to ‘affordable, reliable, sustainable, and modern energy’ is a priority target of one of the sustainable development goals adopted by the United Nations, the number of energy poor around the world remains alarming high (Guan et al. 2023; Pachauri et al. 2021). For example, in 2022 in the European Union, around 42 million households were estimated to be vulnerable to energy poverty, i.e., they faced difficulties keeping their homes at comfortably warm. In these developed countries energy poverty is conditioned by issues of affordability (Vandyck et al. 2023); however, in the developing world energy poverty is more a matter of access to, and the availability of, modern energy services (Pachauri et al. 2021). Regardless of this distinction, energy poverty at the individual level has traditionally been impacted by factors from the triangle of on income, energy efficiency, and energy prices with serious implications for the well-being, health, and social inclusion of affected households (Dobbins et al. 2019; Oliveras et al. 2021). However, the emerging debate is expanding from this traditional triangle to examine the links between energy poverty and new policy areas resulting from climate change and the energy transition (Stojilovska et al. 2022). These problems need to be addressed urgently considering also other elements within the broader energy system (Bessa and Gouveia 2022) especially now that the world finds itself immersed in an unprecedented energy crisis and having to contend with the impact of the COVID-19 pandemic (Carfora et al. 2021; Guan et al. 2023). Failure to address this problem adequately could greatly hinder the achievement of global targets on energy, climate, poverty, and health according to the 2030 Agenda for Sustainable Development (IEA 2023).
To date, and because of the multidimensional nature of energy poverty (Meyer et al. 2018; Halkos and Gkampoura 2021) most of the academic literature has focused its attention on quantifying the phenomenon (Boardman 1991; Faiella and Lavecchia 2021) with a particular focus on the type of indicator being employed (Tirado Herrero 2017; Romero et al. 2018). Recently, academia has begun to show a concern for identifying the determinants of energy poverty (Legendre and Ricci 2015; Costa-Campi et al. 2019), on the understanding that a knowledge of the causes of energy poverty contributes to the proposal of better solutions (Bouzarovski et al. 2012; Stojilovska et al. 2022). Yet, and despite the importance of being able to assess the effectiveness of public policies aimed at reducing energy poverty in the context of the just energy transition, economic evaluations remain scarce. In any case, existing analyses offer only a partial approach to the problem by evaluating a single type of policy (Alvarez and Tol 2021; McCoy and Kotsch 2020; Bagnoli and Bertoméu-Sánchez 2022), overlooking, for the time being, any potential complementarities or overlaps between multiple types of policy. Moreover, recent studies emphasised that siloed approaches to the design of energy poverty policies are not sufficient to address the full scope of this problem (Stojilovska et al. 2022). Here, we seek to further previous studies by examining policy action mechanisms and their interactions in an effort at maximizing their impact on the vulnerable. In the empirical exercise we report here, we assess the hypothetical effectiveness of two types of policies adopted in Spain to reduce energy poverty in terms of both their costs and benefits: we evaluate, on the one hand, policies that act via disposable income, and on a recurring basis, on the bono social—subsidised rates—of electricity and heating and, on the other, policies that act via expenditure, and on a one-off basis, on energy efficiency.
To increase our understanding of the potential effects of these two types of policy action, we drew on existing microdata from the household budget survey (HBS) conducted by Spain’s National Institute of Statistics. To assess the effectiveness of existing energy poverty policies, the magnitude of the phenomena is quantified under scenarios that include alternative policies both individually and in combination.
We find that, on average, the implementation of policies that act via income (i.e. the bono social of electricity and heating) have reduced the number of energy poor households; however, the magnitude of the effect is quite modest, with only 9% of households escaping from the energy poverty trap (i.e. being in a state of energy poverty). In contrast, policies that act via expenditure (i.e. energy efficiency measures) have the potential to minimise between 8%—when targeting more efficient lighting—and 64% of households from energy poverty—when including all the retrofit measures in the dwellings of energy poor households. We further show that when implementing both types of policy (i.e. energy efficiency—total retrofit—and the bono social), it is possible to minimise 67.4% of households from energy poverty. In other words, it is apparent that implementing both policies together results in negligible gains (64% with total retrofit vs. 67.4% with total retrofit + bono social).
This study makes several contributions. One of the standout features of this analysis is the improvement in the line of creating evidence to support the policymaking process. We contribute to the scarce literature on the effectiveness of policy instruments tackling energy poverty (Alvarez and Tol 2021; Bagnoli and Bertoméu-Sánchez 2022). Second, the literature to date has been particularly reliant on evaluating a single type of policy rather than examining potential complementarities between multiple types of policy. However, by leveraging data from Spain, this article enhances previous research by considering jointly income and expenditure policy proposals and their potential impacts in the country. Evaluating the cost-effectiveness of these policies, the paper makes important contributions to the field gaining insights on which support schemes obtain both the highest energy reduction and the highest number of lifted out of energy poverty, especially in times of constrained public budgets. It is worth emphasizing that we assess the theoretical impact of these policies, under the assumption of optimal behaviour and universal coverage for energy-poor households (Al Shawa 2024; Berger and Höltl 2019).
The remainder of the paper is structured as follows. Section 2 consists of a panoramic overview of the energy poverty policies framework. Section 3 presents the methods and Sect. 4 shows our main findings. The last section presents our conclusions and some policy recommendations.
2 Literature Review: Energy Poverty Policy Framework
We analyse the energy poverty policies based on the conceptual framework that classifies policies depending on the mechanism through which these affects the energy poor household. Specifically, there are three main mechanisms: disposable income, energy expenditure, and consumption behaviour (Pye et al. 2017). Traditionally, the main measure used to minimise energy poverty has consisted of income transfers to vulnerable households. Interventions of this type are short-term and palliative in nature, as they seek to provide financial relief to the most vulnerable consumers (in Spain, the so-called bono social falls into this type, given its aim and short-term effects). Indeed, it is evident that countries begin by implementing income measures, as they are the fastest way to address the problem of energy poverty (Kyprianou et al. 2019). The recent increase in energy prices and the impact of the COVID-19 crisis have, once more, shown that the measures implemented to address their effects have been short-term, essentially acting via income (Mastropietro et al. 2020; Hesselman et al. 2021). However, to have a long-term impact, a structural solution to the multidimensional problem is required, one that targets the underlying causes of energy expenditure within the family unit. Indeed, expenditure and behavioural policies with long-term effects are now beginning to gain impetus (Dong et al. 2021; Zhao et al. 2022). For example, in the proposal for a European Union directive on energy efficiency, it is recognised that energy efficiency measures should be given priority when they can alleviate energy poverty (European Commission 2021).
The European Union has set itself the unequivocal goal of achieving an inclusive and fair energy transition (European Commission 2019); however, the specification of the tools to lead the fight against energy poverty remains in the hands of the Member States (Dobbins et al. 2019). Spain is one of the European Union members that has launched the most initiatives (Bouzarovski et al. 2019). Acting through the disposable income of energy poor households, in 2009, the Spanish Government introduced the bono social—a subsidised rate—of electricity, which constitutes a discount applied directly to the consumer’s bill of the so-called voluntary price for the small consumer (or PVPC) tariff. More recently, in 2018, the bono social for heating was introduced as a complementary mechanism to aid vulnerable consumers. This takes the form of a single payment made into the beneficiary’s bank account for the use of heating and hot water (see Methods).
On the expenditure side, Spain implements the PREE Program (Building Energy Rehabilitation), which channels aid to promote action aimed at reducing carbon dioxide emissions, through energy saving, energy efficiency and renewable thermal energies in existing buildings. The program includes improvements to the thermal envelope, the improvement of the energy efficiency of thermal installations (i.e. replacing fossil fuel-based thermal generation facilities with those based on renewable sources and improving the energy efficiency of generation subsystems such as the heat pump), as well as the improved energy efficiency of lighting. A key aspect of the PREE Program is its social scope, with particular attention being given to the granting of aid to carry out rehabilitation actions in buildings that host vulnerable groups or affected by energy poverty. Hence, following the adoption of Spain’s National Strategy against Energy Poverty 2019–2024 (Government of Spain 2019), additional aid is now granted for actions carried out in housing whose owners receive the bono social for electricity.
In general, under the PREE Program, energy efficiency improvements undertaken in entire buildings for all types of reform are granted a subsidy of 35% of the eligible costs, with the exception of lighting installations, for which the subsidy is 15%. Higher subsidised rates are available; for instance, if the household is defined as vulnerable according to the regulations governing the bono social for electricity it qualifies for a 15% higher subsidy, and if the rehabilitation work combines simultaneously two or more elements, one of them being involving the thermal envelope, the household qualifies for a 20% higher subsidy. An additional 15% subsidy can also be obtained in those cases where the efficiency target is met, that is, when a dwelling achieves an A or B energy rating.
Depending on the type of policy implemented—income vs. expenditure—the effect on the group trapped in energy poverty is likely to differ. According to the expenditure-based indicator of low income-high costs (LIHC) (Hills 2012), a household is defined as energy poor if its income falls below a certain poverty threshold (i.e. 60% of the median income) and its energy expenses climb above a certain energy threshold (the equivalent median of energy expenditure calculated on the total number of households) (see Fig. 1). Thus, if a household is in the lower left quadrant, it will be considered energy poor. This household can escape from energy poverty in one of three ways: (1) by increasing the disposable income, for example by means of transfers from Spain’s bono social for electricity or heating, (2) by decreasing energy costs thanks to the operation of an expenditure policy that improves the energy efficiency of the house, or (3) by both increasing income and decreasing energy costs thanks to combination of both income and expenditure policies. The LIHC indicator has been selected to evaluate the impact of the policies on the energy poverty rate (see Sect. 3.4).
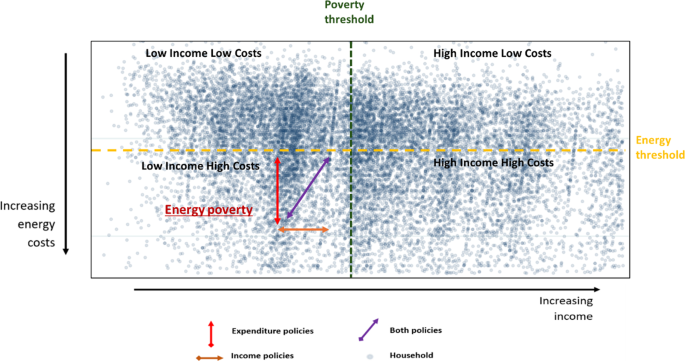
Source: Based on Hills (2012)
Definition of energy poverty and sample distribution (pre-policies).
3 Methods
In what follows, we explain how the various policies targeting energy poverty are replicated in the 19,868 households of 2019 HBS database. This database is carried out by the Spanish National Statistics Institute and provides data at the household level on energy expenditure and a wide variety of socioeconomic variables. Our aim is to exploit the household characteristics available in that database to simulate policy implementation and to assess their potential impacts at the household level. As discussed, we consider two types of policy: income versus expenditure.
3.1 Income Policy—Bono Social for Electricity (BSE) Measure
Here, we describe the replication of the bono social for electricity (henceforth BSE). The first step involves the identification of ‘vulnerable’ and ‘severely vulnerable’ households in the microdata. The identification of these target households is based primarily on household income levels, but other elements must also be considered. Income thresholds are defined in reference to the Indicador Público de Renta de Efectos Múltiples or IPREM, an indictor used in Spain as a benchmark for the granting of aid, subsidies, or unemployment benefit (for example, in 2019 the IPREM index for 14 payments was 1520€ per year). More specifically, the income thresholds that define a ‘vulnerable’ household are:
-
1.
1.5 * the IPREM index of 14 payments, in the event that it is not part of a family unit or there is no minor in the family unit;
-
2.
2 * the IPREM index of 14 payments, in the event that there is a minor in the family unit; and
-
3.
2.5 * the IPREM index of 14 payments, in the event that there are two minors in the family unit.
The BSE definition of a ‘vulnerable’ household incorporates two extra conditions, independent of the those defined by income thresholds:
-
1.
Being recognised as a familia numerosa or large family, understood to be one or two parents living with three or more children, whether or not the children are common to both parents.
-
2.
The signatory of the electricity contract (or all the household members that have an income but who are not receiving other income whose annual aggregate amount exceeds 500 euros) is a pensioner of the social security system. There are many variations of the minimum pension level depending on the household composition. We employ the minimum retirement income by category and identify households according to their composition.
We also identify those households that meet the stricter requirements for being recognised as ‘severely vulnerable’ households. A ‘severely vulnerable’ household is one that receives an annual income below 50% of the threshold established for being considered a ‘vulnerable’ consumer. If the household constitutes a familia numerosa, the family unit must be in receipt of an annual income ≤ 2 × IPREM of 14 payments. Additionally, if all the members who have income in the family unit receive a minimum pension, and do not receive other income whose annual aggregate amount exceeds 500 euros, the household unit must receive an annual income ≤ 1 × IPREM of 14 payments to be considered ‘severely vulnerable’.
Finally, less stringent income criteria are reserved for special cases. Particularly, if special circumstances are met, the established limits are increased by 0.5 IPREM points. Special cases include criteria that we have been able to replicate in the database—for example, single-parent families—and others that are impossible to replicate due to data limitations—for example, victims of gender violence or terrorism, and the disabled.
The procedure outlined above allows us to identify the ‘vulnerable’ households that meet the requirements to benefit from the policy; however, the actual number of BSE beneficiaries is significantly lower. The main reasons for this are that BSE is only for consumers under the regulated tariff (PVPC), many households have either not been informed about the BSE, are unaware they have the right to access the subsidy, or do not know how to complete the bureaucratic process to become a beneficiary. In our empirical evaluation, to assess the full potential of policies tackling energy poverty, we assume that the measure reaches all vulnerable households.
Having identified the ‘vulnerable’ and ‘severely vulnerable’ households, we applied the income transfer (equivalent to the corresponding discount) to their electricity bills—i.e. a 25% discount for vulnerable households and a 40% discount for ‘severely vulnerable’ households. Thus, we calculate (Eq. 1) the income transfer from the BSE in each household i as:
The last element of the BSE replicated in our assessment is ensuring that the income transfer is limited to certain levels of electricity consumption. For instance, families without children have a consumption limit of 1380 kWh per year, and any consumption above this limit is not discounted (at 25 or 40% depending on the case). The data show that in 2019, 10.65% of ‘vulnerable’ consumers exceeded the established consumption limits and that, therefore, these limits were binding for the total consumption of these households. To replicate these limits in our calculations, the consumption thresholds were transformed from kWh to EUR based on retail electricity price data and applied to the BSE discounts.
3.2 Income Policy—Bono Social for Heating Measure
The bono social for heating is assigned automatically when a household is a recipient of the BSE.Footnote 1 Thus, in order to identify the target households, we use the same requirements as outlined above for the BSE. However, the amount transferred is determined according to the climate zone in which the household is located (see Fig. 7). The implementation of these climate zones is the main challenge faced when seeking to replicate this measure in our database. While the regulations state that climate zones are assigned at a municipal level, due to database limitations, we are obliged to assign the climate zones by the corresponding Autonomous Community or regional NUTS-2 level (Eurostat 2016). Specifically, for each Community we calculate the average bono social for heating according to the climate zones present in each territory and in this way, we determine the amount transferred to each household (\({Heatingbonus}_{i})\).
Finally, to analyse energy poverty rates before and after the application of these policies, we have to compute respective household income levels (Eq. 2). The household income before policy implementation is expressed as \({(Inc}_{i}^{o})\), while household income after the bono social is applied is expressed as \({(Inc}_{i}^{b})\), where:
3.3 Expenditure Policy—Energy Efficiency Measures
Here we provide detailed information about the use of HBS data (specifically as regards dwelling characteristics) to replicate the impact of different rehabilitation measures (that is, those included in the energy efficiency measure) in terms of household costs and savings.
The methodology used to calculate theoretical energy efficiency improvements at the household level is highly detailed and because we only have information about housing type (apartment, townhouse, etc.), surface area (\({m}^{2}\)) and the number of rooms in the dwelling, we are only able to replicate this in part in our database. We build on the findings of a previous technical document (Capdevila et al. 2012). This allows us to characterize, in a comparable and consistent fashion, a wide set of Institute for the Diversification and Saving of Energy (IDAE)-PREE Program eligible retrofit works with the limited information on dwelling characteristics in the database. To the best of our knowledge, this is the only study or technical document available to meet our purposes here, with the added advantage of providing retrofit results tailored to Spanish dwellings and taking into consideration the wide climate variations in Spain, along with their impact on energy efficiency. Specifically, we use the sustainability parameters for a set of energy efficiency rehabilitation works for Spanish dwellings. For each energy efficiency work type, we use the improvement in energy usage in kWh/m2 year of final energy consumption to calculate the current value of future gains from the energy efficiencyFootnote 2—denoted as \({Eff\_gains}_{i}^{w}\left(\frac{\text{kWh}}{{\text{m}}^{2}}\right)\), and the cost of implementation (€/m2)—denoted as \({Cost}^{W}\left(\frac{EUR}{{\text{m}}^{2}}\right)\). The retrofit works considered refer to the rehabilitation of the envelope (facade insulation, cover insulation and insulation holes), equipment (condensation boiler, efficient boiler, solar thermal and heat pump including cooling and heating technologies), and lighting.
We calculate the final annual investment cost after subsidies for the different energy efficiency works that each household undertakes. First, in order to calculate the annual investment cost of each energy efficiency intervention, we include the lifespan of the different works and equipment.Lifespan corresponds to the typical lifespan of the given equipment (see Nägeli et al. 2019; Košičan et al. 2021). We assume the following lifespans (in years): facade and gaps—30, roof—20, solar thermal—20, other equipment—12.5, and lighting—10. Then, we define the final annual cost (in €) of the energy efficiency work \(w\) for the household \(i\) as \({Cost}_{i}^{w}\). All the different retrofit improvements are considered individually and also in combination. The interventions are evaluated for ‘vulnerable’ and ‘non-vulnerable’ consumers. The percentage of eligible costs depends on the type of intervention and is assigned according to the standards established in the program design (as detailed in the measure description in the “Bono social vs. energy efficiency” section).
Mathematically, our costs and savings model can be summarised in the following equations (Eq. 3.1 and Eq. 3.2). The following investment costs are assigned at the household level for the different interventions.
For lighting retrofits only, the \({Cost}_{i}^{w}\) is equal to:
Otherwise \({Cost}_{i}^{w}\) is equal to:
The mean annual investment costs of the different rehabilitation measures, obtained by application to our database (as detailed above), are summarised in Table 1.
The energy efficiency gains (in €, Eq. 4) resulting from the energy efficiency work \(w\) for household \(i\), are defined as:
Therefore, the monetary savings (Eq. 5) obtained from the efficiency work \(w\) by household \(i\), are:
Finally, we subtract the savings from the household’s energy expenditures to know the impact of the measure in terms of energy expenditure. Where \({EE}_{i}^{o}\) is the energy expenditure of household \(i\) before any measure has been applied, and \({EE}_{i}^{w}\) is the energy expenditure of household \(i\) after the energy efficiency measure \(w\) has been applied.
In short, we simulated the impact of the energy efficiency retrofit measure \(w\) on the household’s energy consumption. Starting with a household’s energy consumption, we subtract the energy efficiency gains obtained thanks to the retrofit work and add the annual cost of the retrofit after applying the PREE Program subsidies.
3.4 Impact of Policies on Energy Poverty
The final step in our methodology is to evaluate the impact of the policies on the energy poverty rate. To characterize energy poverty, we use the LIHC indicator, which defines a household as energy poor when its energy costs are above the national median and its residual income after energy expenditure is below 60% of the median residual income or the poverty line.Footnote 3 Following Romero et al. (2018), who took their lead from Hills (2012), the median of energy expenditure is subtracted from the median household income to be consistent with the first term of the equation. In addition, with this formulation, we also overcome the main criticism made by Robinson et al. (2018) regarding the consideration of the median fuel cost instead of 60% of the median as in the case of income.
The household energy expenditure and income data come from the Spanish HBS. They are treated in order that the UC can be considered. These data are used in the following variables:
-
\(EE_{i}\) is the observed energy expenditure of household i
-
\(\widetilde{EE}_{{}} {\text{ is the}}\) observed country median energy expenditure
-
\(Inc_{i}\) is the observed income of household
-
\(\widetilde{Inc}_{{}}\) is the observed country median income
Using the survey information, we can calculate energy poverty before the benefits of the measure are felt by households.
Hence, before the bono social uses or energy efficiency policies have been applied, a household is energy poor if:
Our goal is to understand the impact of the bono social on Spanish energy poverty. As explained above, we have defined household income before the bono as \({Inc}_{it}^{o}\) while \({Inc}_{it}^{b}\) represents household income after the selected household receive the bono social. Hence, after the bono has been received, a household is energy poor if:
Analogously, based on energy expenditure before policies as \({EE}_{i}^{o}\), we can define the energy expenditure of household \(i\) in period \(t\) after the energy retrofit measure w has been applied as \({EE}_{i}^{w}\). Hence, a household \(i\) in period \(t\) is poor after retrofit work \(w\) if
Hence, if both the bono social and the retrofit policies are applied, energy poverty is defined as:
As explained before, we use the LIHC indicator of energy poverty for our analysis. This methodological choice is deemed suitable, given the nature of the study with an individual approach to, both, defining the problem and performing the policy assessment, and also given the availability of relevant data. The ideal empirical approach to the research question we are facing would potentially imply the use alternative indicators of energy poverty. Also, it could be of interest to incorporate broader definition of energy poverty to consider factors beyond the individual ones in defining the energy poverty situation and contextualize it within the broader energy system, where relevant elements, like the energy prices, could be directly incorporated into the analysis. Although combining indicators is desirable to measure broader aspects, it implies challenges such as database availability and comparability.
At the moment, in spite of there not being any absolute consensus about the best metric for the analysis of energy poverty, since no measurement is perfect (Sareen et al. 2020), it is possible to affirm that there is a subset of indicators that, from the perspective of income and/or expenditure, allows the problem to be robustly quantified, and therefore, ultimately, solutions to be put forward for public policy. This subset is made up of the indicators that are most frequently used in the literature, and which have been those favoured mainly by the United Kingdom government, and in recent years they have also gained ground in research based in the EU (Legendre and Ricci 2015; Bouzarovski and Tirado Herrero 2017).
Measurement through the LIHC is not free of criticisms. One of the issues frequently pointed out is that, since the indicator defines poverty as those who earn 60% of median income in combination with the median energy cost (instead of 60% of the median energy cost), this approach excludes single person households (Broadman 2012; Robinson et al. 2018). In our analysis this limitation is overcome by using the 60% of the median for both, income and energy expenditure. It is also claimed that the LIHC indicator tends to prioritise energy efficiency as a solution to fuel poverty, distracting from other drivers more related to affordability (Middlemiss 2016). This is not a major source of concern given that the nature of our study is beyond the simple statistical analysis of the indicator, with the assessment of the potential effects from alternatives policies, including both income and expenditure side of the analysis.
No indicator of energy poverty is likely to be perfect, but the LIHC includes those on the margin of poverty who are pushed to energy poverty by their high energy requirement, hence considering the problem from both income and cost perspectives (Hills 2011). Grounded on the above but acknowledging the limitations of using the LIHC as a single measurement, this study relies on a modified LIHC indicator to quantify the energy poverty in Spain.
Finally, it is relevant to mention that structural elements of the energy model which may contribute to energy poverty could be relevant to contextualize the phenomenon and enrich the analysis. However, the policy assessment performed in this study is based on simulations using data for a single year, in a system with uniform energy pricing. This implies that, in the policy simulations, the only changes faced by energy poor households are those coming from the policy itself, while the structural elements are assumed to remain constant, i.e. ceteris paribus. While this approach could be considered too narrow and ideally additional elements could be incorporated to provide a more comprehensive view of the energy poverty problem, actually allows to provide a straightforward answer to the research question on policies acting though alternatives mechanism, directly affecting the energy poverty condition at the household level (income and energy expenditure).
4 Results: Impact Assessment
The richness of our composition of the data makes it possible to design scenarios that consider the households’ socioeconomic characteristics, and to simulate policy implementation and their impacts at the household level. The policy evaluation presented is based on results using data for the year 2019, which included the bono social applied before the pandemic crisis, given that temporary modifications were introduced with the onset of the pandemic.
We analyse the results with regards to two specific dimensions: first, in terms of the resultant fall in energy expenditure (and its equivalent in the case of an income transfer); and second, in terms of the households lifted out of the energy poverty trap. Table 2 provides details on both energy poor households and all households in the sample (19,868 households), about the pre-policy (original) values and the outcomes following the implementation of the different measures (energy efficiency and bono social). Regarding pre-policy values, our data show that 6.8% of the total sample of households experienced energy poverty, according to the LIHC indicator. Moreover, the average yearly income and energy expenditure per household of the overall population stood at 25,139€ and 1076€, while the comparative figures for the energy poor households stood at 10,421€ and 1481€, respectively.
Regarding the evaluation of policies in terms of their impact on energy expenditure, the results for the effects of the energy efficiency measure highlight that, in the case of actions impacting the thermal envelope, all leads to the similar reduction in energy expenditure, decreasing outlay by an average 6% in the case of energy poor households and by 7% when applied to all households in the sample. In the case of actions to improve equipment, the installation of a condensation boiler leads to the greatest reduction in energy expenditure, with an average 16% decrease in poor households and 20% in all households. Lighting is the energy efficiency measure that has the lowest impact on expenditure, being responsible for a 3 and 4% fall when installed in poor households and in all households, respectively. Finally, combining retrofit solutions leads to lower levels of energy expenditure than implementing individual solutions (thus, all thermal envelope actions lead to a 17% reduction, all equipment actions to a 23% reduction, and total retrofit reduces expenditure by 39% in energy poor households). Overall, our results show that the energy efficiency gains are always smaller for energy poor households, which would appear to reflect the smaller size of these dwellings in the sample, giving them a lower potential gain. However, in relative terms, when considering the saving with respect to the average income, the energy poor households would obtain the highest potential gains. According to our results, the bono social measure leads to an increase in average disposable income of 280€ with respect to the pre-policy income of energy poor households. This transfer means their disposable income is 2.6% higher, which—if spent on energy is equivalent to a 19% reduction of the energy expenditure. If we combine this income transfer and all the energy efficiency measures (i.e. total retrofit), then the impact is equivalent to a 57% decrease in the energy expenditure of energy poor households.
In addition to evaluating these policies in terms of their impact on energy expenditure, it is also critical to assess their effectiveness in terms of the proportion of households that escape the energy poverty trap. In this regard, our estimates show that improvements to the thermal envelope have the potential of saving 16% of households from energy poverty in the case of facade insulation, cover insulation and insolation of holes, leading to shares of energy poverty after the measure of 5.7%. Meanwhile, improvements to equipment have the potential of saving 36% of households from the poverty trap when the condensation boiler is replaced and 8% with more efficient lighting, leading to shares of energy poverty after the measure of 4.3% and 6.2%, respectively (see Fig. 2).
One of the most relevant results from this simulation is found when under the energy efficiency measure all retrofit options are combined (see Fig. 3). Thus, when energy poor households adopt all the retrofit measures, 64% can potentially be lifted out from the energy poverty trap, leading to an energy poverty share of only 2.4% after the measure is implemented.
If we compare all the effects of energy efficiency measures combined with an income policy on the proportion of households that escape the energy poverty trap, our outcomes for the bono social are considerably inferior. Indeed, bono social of electricity and heating only have the potential of saving 9% of households from energy poverty, with a share of energy poverty after the policy equals to 6.2% (similar to pre-policy share of 6.8%) (see Fig. 4).
Finally, when the energy poor households implement all possible policies considered in this study, including, that is, all the energy efficiency (total retrofit) measures and the bono social, our results indicate that this has the potential of saving 67.4% households from the energy poverty trap, achieving a share of energy poverty after the policy of only 2.2% (see Fig. 5). If we compare this result with that of the impact of a total retrofit, it is apparent that the gain from also introducing the bonos, in terms of the reduction in energy poverty, is extremely small (64% with total retrofit vs. 67.4% with total retrofit + bonos), leading to similar shares of energy poverty (2.4% with total retrofit vs. 2.2% with total retrofit + bonos). Energy efficiency is widely recognized within the European Union as a transformative solution to alleviate energy poverty. However, it is important to consider potential obstacles to the effectiveness of building renovation and thermal insulation efforts, such as the well-documented rebound effect (see Berkhout et al. 2000; Sorrell and Dimitropoulos 2008). The energy efficiency literature has studied this effect on energy-poor consumers, indicating that the benefits of efficiency interventions can be diminished by behavioural responses aimed at increasing thermal comfort (Berger and Höltl 2019; Milne and Boardman 2000). To mitigate these challenges, energy efficiency measures must be complemented by behavioural initiatives that aim to modify energy consumption habits through training and personalized advice. The overarching goal is to transition from established pre-retrofit practices and associated energy use patterns to new, more efficient practices across various aspects such as lighting, appliance usage, heating systems, and more (Rau et al. 2020).
Additional simulations were conducted to explore the sensitivity of our results with respect to the sample year used and the LIHC threshold. Firstly, we analysed results using data from the year 2019, as this included the bono social applied before the pandemic crisis, avoiding the temporary modifications introduced to address this shock. We also performed simulations with data from the most recent year available, 2022, when the energy poverty rate increased to 7.7% (up from 6.8% in 2019). While the bono social discounts in 2019 were 25% or 40%, depending on the level of vulnerability, these discounts increased to 65% and 80% following the implementation of the post-pandemic social shield. Results for the impact of energy efficiency in 2022 align with those from 2019, and as expected, there are considerable differences in terms of the bono social impact. Specifically, the extensive bono social for electricity and heating has the potential to save 24.7% of households out of energy poverty, resulting in a post-policy energy poverty rate of 5.8% (compared to 9% of households saved of energy poverty in 2019, with a post-policy rate of 6.2%). Given that this extensive measure is planned to be removed by the end of 2024, the results for 2019, which reflect a more stable context, remain the focus of our analysis.
Secondly, following previous studies, we used the energy poverty measure defined by the LIHC, with the poverty threshold set at 60% of the median income. Despite being a standard, this threshold can be considered somewhat arbitrary, and a sensitivity analysis helps to understand the significance of this convention. While the results are slightly sensitive to changes in the threshold, the main conclusions remain unaffected, as the order of magnitude stays consistent across different policies implemented. Figure 6 presents the simulation results in terms of the percentage of households lifted out of energy poverty after the policies, considering three alternative LIHC thresholds.
5 Conclusions
Our analysis provides new insights into how the energy poverty level might be alleviated by combining policies based on income (bono social) and expenditure (energy efficiency) tools. The results of our empirical evaluation have a number of highly relevant policy considerations. First, both income and expenditure policies have the potential to reduce the proportion of energy poor households in an economy; however, the magnitude of their respective effects differs greatly. The average impact of energy efficiency measures provides for a greater reduction in the number of energy poor households than the resource transfer measures, where the effect is quite modest. Yet, our results show the outcomes of energy efficiency measures to be highly heterogeneous depending on the type of actions implemented, with the installation of efficient lighting having the smallest impact and the rehabilitation of a building’s thermal envelope having the greatest impact.
Second, and of considerable relevance to our discussion here, is the finding that the combination of energy efficiency measures has better outcomes than the implementation of single energy efficiency measures. Third, the greatest reduction in energy poverty is obtained when income and expenditure measures are jointly applied. However, the incremental effect of the income policy once total retrofit measures has been implemented is highly marginal, reducing the energy poverty level achieved by less than one per cent.
Our outcomes provide useful arguments for the debate regarding society’s policy costs to combat energy poverty, we contribute to this by comparing the total annual expenditure of each measure. The total expenditure of the bono social, assuming universal coverage, is calculated by adding the annual cost of the corresponding benefit to each energy poor household. Analogously, the expenditure of total retrofit, is the result of adding the annualised cost of implementing the measures, assuming universality among the poor household. As a result, when implementing income policies with the amount of EUR 936 million each year, we find that only 9% of households would escape the energy poverty trap. These estimates are an order of magnitude higher than the estimated EUR 926 million of annual average expenditure needed to implement full retrofit measures, which would potentially allow 64% of households to escape the poverty trap, assuming optimal behaviour of consumers and access to finance.
Overall, we can conclude that, while both types of policy are effective at reducing the number of energy poor households, income policies do not break the vicious cycle of energy poverty. Rather, placing energy efficiency programs at the heart of policies to combat energy poverty constitutes a better long-term solution. However, policymakers should be aware that there are factors may influence the effectiveness of these policies. For example, while the bono social of electricity in Spain has shown significant improvements since its inception, there are areas that still require attention. Specifically, it is crucial to simplify the application process further (including necessary documentation and adjusting language to enhance understanding for interested groups). In addition, we still have much to learn about how energy efficiency measures can reach the most vulnerable households. This will clearly require greater commitment and research on how best to address the financial obstacles to the uptake of energy efficiency measures, especially for low-income households. While financial barriers are significant, there are other obstacles to consider, such as the lack of awareness about renovation policies, split incentives between owners and tenants, mistrust of renovation providers, and the presence of cumbersome and slow administrative processes for aids, particularly affecting low-income families with limited education. Therefore, energy efficiency policies must be complemented by the creation of one-stop shops that offer not only financing solutions but also personalized technical advice, and support to households throughout all the renovation process.Please note that the Figure 2 has been changed to figure 7, Figure 3 has been changed to figure 2, Figure 4 has been changed to figure 3, Figure 5 has been changed to figure 4, Figure 6 has been changed to figure 5, Figure 7 has been changed to figure 6 and also the citations are changed in text. Please check and confirm.The new numbering of the figures creates confusion for the reader. It is possible that the figure appearing in the appendix has the following name Fig. A.1 or similar.
The preceding analysis has provided useful insights into the factors influencing energy poverty and the potential impacts of different policy interventions. However, we should mention a number of limitations that future research might address. First, to our ambitious goal, although we could only explain two policies in detail, we focus on the most relevant policies in the Spanish context. We are aware that the narrow scope of the study means that important insights may be missed by not considering a wider range of policies, for instance, behavioural policies, but they are not directly comparable (heterogeneous in terms of targets, resources, and mechanisms). In order to do that, public authorities should extend data availability about actions related with energy poverty in order to better measure the incidence and better design policy recommendations. Second, the energy efficiency policies analysed still fail to cover the costs for numerous vulnerable families, who must pay the costs upfront while financial aid is often only provided afterwards. This creates barriers for households seeking to access these measures. Further research should be conducted to understand in the financing strategy and the total costs of the policy in order to provide a more comprehensive evaluation process.
Notes
Given that the bono social is made up two bundled elements, and in fact, the thermal bono is a transfer (in a strict sense), the methodological decision was to treat both as a single transfer. This is because, in practical terms, they allow for a greater disposable income.
Following the recommendations by ECEEE and Ecofys (2015) on discount rates for energy efficiency projects by households, we use a 3% discount rate for the net present value computed to obtain the current value of future gains from energy efficiency.
Another relevant indicator that aligns with our analysis is the Low Income Low Energy Efficiency (LILEE) indicator, commonly used in the United Kingdom. However, due to data availability, it is not feasible to calculate this indicator for our Spanish sample (Government of United Kingdom 2024).
References
Al Shawa B (2024) Keeping the rebound and energy poverty at bay: a novel tariff structure for the United Kingdom’s residential sector. Energy Res Soc Sci 112:103508. https://doi.org/10.1016/j.erss.2024.103508
Alvarez GG, Tol RSJ (2021) The impact of the bono social de electricidad on energy poverty in Spain. Energy Econ 103:105554. https://doi.org/10.1016/j.eneco.2021.105554
Bagnoli L, Bertoméu-Sánchez S (2022) How effective has the electricity social rate been in reducing energy poverty in Spain? Energy Econ 106:105792. https://doi.org/10.1016/j.eneco.2021.105792
Berger T, Höltl A (2019) Thermal insulation of rental residential housing: do energy poor households benefit? A case study in Krems, Austria. Energy Policy 127:341–349. https://doi.org/10.1016/j.enpol.2018.12.018
Berkhout PH, Muskens JC, Velthuijsen JW (2000) Defining the rebound effect. Energy Policy 28(6–7):425–432. https://doi.org/10.1016/S0301-4215(00)00022-7
Bessa S, Gouveia JP (2022) A framework for policy mix analysis: assessing energy poverty policies. J Environ Econ Policy. https://doi.org/10.1080/21606544.2022.2153744
Boardman B (1991) Fuel poverty: from cold homes to affordable warmth. Belhaven Press, London
Boardman B (2012) Fuel poverty synthesis: lessons learnt, actions needed: fuel poverty comes of age: commemorating 21 years of research and policy. Energy Policy 49:143–148. https://doi.org/10.1016/j.enpol.2012.02.035
Bouzarovski S, Tirado Herrero S (2017) The energy divide: Integrating energy transitions, regional inequalities and poverty trends in the European Union. Eur Urban Reg Stud 24(1):69–86. https://doi.org/10.1177/0969776415596449
Bouzarovski S, Petrova S, Sarlamanov R (2012) Energy poverty policies in the EU: a critical perspective. Energy Policy 49:76–82. https://doi.org/10.1016/j.enpol.2012.01.033
Bouzarovski S, Thomson H, Cornelis M, Rogulj I, Campuzano M, Goermaere S (2019) Transforming energy poverty policies in the European Union: second annual report of the European Union Energy Poverty Observatory. EU Energy Poverty Observatory: Manchester, 20–01
Buzar S (2016) Energy poverty in Eastern Europe: hidden geographies of deprivation. Routledge, London
Capdevila I, Linares E, Folch R (2012) Eficiencia energética en la rehabilitación de edificios. Energía y medio ambiente 25. Fundación Gas Natural Fenosa
Carfora A, Scandurra G, Thomas A (2021) Forecasting the COVID-19 effects on energy poverty across EU member states. Energy Policy. https://doi.org/10.1016/j.enpol.2021.112597
Costa-Campi MT, Jové-Llopis E, Trujillo-Baute E (2019) Energy poverty in Spain: an income approach analysis. Energy Sources Part B Econ Plan Policy 14(7–9):327–340. https://doi.org/10.1080/15567249.2019.1710624
Dobbins A, Fuso Nerini F, Deane P, Pye S (2019) Strengthening the EU response to energy poverty. Nat Energy 4(1):2–5. https://doi.org/10.1038/s41560-018-0316-8
Dong K, Ren X, Zhao J (2021) How does low-carbon energy transition alleviate energy poverty in China? A nonparametric panel causality analysis. Energy Econ 103:105620. https://doi.org/10.1016/j.eneco.2021.105620
ECEEE and Ecofys (2015) Evaluating our future. The crucial role of discount rates in European Commission energy system modelling. European Council for an Energy Efficient Economy. www.eceee.org/policy-areas/discount-rates/
European Commission (2019) Communication from the Commission to the European Parliament, the European Council, the Council, the European Economic and Social Committee and the Committee of the Regions. The European Green Deal. Brussels, 11.12.2019. COM(2019) 640 final. Retrieved from https://eur-lex.europa.eu/legal-content/EN/TXT/?uri=CELEX%3A52019DC0640. Accessed Dec 2021
European Commission (2021) Proposal for a Directive of the European Parliament and of the Council on energy efficiency (recast). Brussels, 14.7.2021. COM(2021) 558 final. 2021/0203(COD). https://eur-lex.europa.eu/legal-content/EN/TXT/?uri=CELEX%3A52021PC0558. Accessed Dec 2021
Eurostat (2016) Statistics Illustrated-NUTS classification (Nomenclature of territorial units for statistics) according to NUTS 2016. https://ec.europa.eu/eurostat/web/nuts/statistics-illustrated. Accessed May 2022
Faiella I, Lavecchia L (2021) Energy poverty. How can you fight it, if you can’t measure it? Energy Build 233:110692. https://doi.org/10.1016/j.enbuild.2020.110692
Government of Spain (2019) Ministry for the ecological transition and the demographic challenge (MITECO).national strategy against energy poverty 2019–2024. https://www.miteco.gob.es/es/prensa/estrategianacionalcontralapobrezaenergetica2019-2024_tcm30-496282.pdf. Accessed June 2022
Government of United Kingdom (2024) Fuel poverty methodology handbook (Low Income Low Energy Efficiency). https://assets.publishing.service.gov.uk/media/65ccf6341d9395000c9466a7/fuel-poverty-methodology-handbook-2024.pdf. Accessed April 2024
Guan Y, Yan J, Shan Y, Zhou Y, Hang Y, Li R, Liu Y, Liu B, Nie Q, Bruckner B, Feng K, Hubacek K (2023) Burden of the global energy price crisis on households. Nat Energy 8:304–316. https://doi.org/10.1038/s41560-023-01209-8
Halkos GE, Gkampoura EC (2021) Evaluating the effect of economic crisis on energy poverty in Europe. Renew Sustain Energy Rev 144:110981. https://doi.org/10.1016/j.rser.2021.110981
Hesselman M, Varo A, Guyet R, Thomson H (2021) Energy poverty in the COVID-19 era: mapping global responses in light of momentum for the right to energy. Energy Res Soc Sci 81:102246. https://doi.org/10.1016/j.erss.2021.102246
Hills J (2011) Fuel poverty: the problem and its measurement. Centre for Analysis of SocialExclusion, London
Hills J (2012) Getting the measure of fuel poverty: Final Report of the Fuel Poverty Review. CASEreport, 72. Centre for Analysis of Social Exclusion, London School of Economics and Political Science, London
IEA (2023) Tracking SDG7: The energy progress report, 2023, IEA, IRENA, UNSD, World Bank, WHO, Paris. https://www.iea.org/reports/tracking-sdg7-the-energy-progress-report-2023. Accessed April 2024
Košičan J, Pardo Picazo MÁ, Vilčeková S, Košičanová D (2021) Life cycle assessment and economic energy efficiency of a solar thermal installation in a family house. Sustainability 13:2305. https://doi.org/10.3390/su13042305
Kyprianou I, Serghides DK, Varo A, Gouveia JP, Kopeva D, Murauskaite L (2019) Energy poverty policies and measures in 5 EU countries: a comparative study. Energy Build 196:46–60. https://doi.org/10.1016/j.enbuild.2019.05.003
Legendre B, Ricci O (2015) Measuring fuel poverty in France: which households are the most fuel vulnerable? Energy Econ 49:620–628. https://doi.org/10.1016/j.eneco.2015.01.022
Mastropietro P, Rodilla P, Batlle C (2020) Emergency measures to protect energy consumers during the Covid-19 pandemic: a global review and critical analysis. Energy Res Soc Sci 68:101678. https://doi.org/10.1016/j.erss.2020.101678
McCoy D, Kotsch RA (2020) Quantifying the distributional impact of energy efficiency measures. Energy J 42(6):121–144
Meyer S, Laurence H, Bart D, Middlemiss L, Maréchal K (2018) Capturing the multifaceted nature of energy poverty: lessons from Belgium. Energy Res Soc Sci 40:273–283. https://doi.org/10.1016/j.erss.2018.01.017
Middlemiss L (2016) A critical analysis of the new politics of fuel poverty in England. Crit Soc Policy 37(3):425–443. https://doi.org/10.1177/0261018316674851
Milne G, Boardman B (2000) Making cold homes warmer: the effect of energy efficiency improvements in low-income homes A report to the energy action grants agency charitable trust. Energy Policy 28(6–7):411–424. https://doi.org/10.1016/S0301-4215(00)00019-7
Comisión Nacional de los Mercados y la Competencia (CNMC) (2020) Boletín de indicadores eléctricos de abril 2020. Publishing CNMC. https://www.cnmc.es/listado/sucesos_energia_mercado_electrico_boletines_de_indicadores_electricos/block/250 Accessed April 2020
Nägeli C, Farahani A, Österbring M, Dalenbäck JO, Wallbaum H (2019) A service-life cycle approach to maintenance and energy retrofit planning for building portfolios. Build Environ 160:106212. https://doi.org/10.1016/j.buildenv.2019.106212
Oliveras L, Peralta A, Palència L, Gotsens M, López MJ, Artazcoz L, Borell C, Marí-Dell’Olmo M (2021) Energy poverty and health: trends in the European Union before and during the economic crisis, 2007–2016. Heal Place 67:102294. https://doi.org/10.1016/j.healthplace.2020.102294
Pachauri S, Poblete-Cazenave M, Aktas A, Gidden MJ (2021) Access to clean cooking services in energy and emission scenarios after COVID-19. Nat Energy 6(11):1067–1076. https://doi.org/10.1038/s41560-021-00911-9
Pye S, Dobbins A, Baffert C, Brajković J, Deane P, De Miglio R (2017) Energy poverty across the EU: analysis of policies and measures. In: Welsch M (ed) Europe’s energy transition: insights for policy making. Academic Press, London, pp 261–280. https://doi.org/10.1016/B978-0-12-809806-6.00030-4
Rau H, Moran P, Manton R, Goggins J (2020) Changing energy cultures? Household energy use before and after a building energy efficiency retrofit. Sustain Cities Soc 54:101983. https://doi.org/10.1016/j.scs.2019.101983
Robinson C, Bouzarovski S, Lindley S (2018) Getting the measure of fuel poverty: the geography of fuel poverty indicators in England. Energy Res Soc Sci 36:79–93. https://doi.org/10.1016/j.erss.2017.09.035
Romero JC, Linares P, López X (2018) The policy implications of energy poverty indicators. Energy Policy 115:98–108. https://doi.org/10.1016/j.enpol.2017.12.054
Sorrell S, Dimitropoulos J (2008) The rebound effect: microeconomic definitions, limitations and extensions. Ecoll Econ 65(3):636–649. https://doi.org/10.1016/j.ecolecon.2007.08.013
Stojilovska A, Guyet R, Mahoney K, Gouveia JP, Castaño-Rosa R, Živčič L, Barbosa R, Tkalec T (2022) Energy poverty and emerging debates: beyond the traditional triangle of energy poverty drivers. Energy Policy 169:113181. https://doi.org/10.1016/j.enpol.2022.113181
Tirado Herrero S (2017) Energy poverty indicators: a critical review of methods. Indoor Built Environ 26(7):1018–1031. https://doi.org/10.1177/1420326X17718054
Vandyck T, Della Valle N, Temursho U, Weitzel M (2023) EU climate action through an energy poverty lens. Sci Rep 13(1):6040. https://doi.org/10.1038/s41598-023-32705-2
Zhao J, Dong K, Dong X, Shahbaz M (2022) How renewable energy alleviate energy poverty? A global analysis. Renew Energy 186:299–311. https://doi.org/10.1016/j.renene.2022.01.005
Acknowledgements
The authors acknowledge support from project PID2022-140546OB-I00 funded by MCIU/AEI/https://doi.org/10.13039/501100011033 and ERDF, EU; from project 2021SGR00355 funded by the Departament de Recerca i Universitat de la Generalitat de Catalunya, and from the Chair of Energy Sustainability (IEB, Universitat de Barcelona).
Funding
Open Access funding provided thanks to the CRUE-CSIC agreement with Springer Nature.
Author information
Authors and Affiliations
Corresponding author
Ethics declarations
Conflict of interest
The authors have no competing interests to declare that are relevant to the content of this article.
Additional information
Publisher's Note
Springer Nature remains neutral with regard to jurisdictional claims in published maps and institutional affiliations.
Appendix
Rights and permissions
Open Access This article is licensed under a Creative Commons Attribution 4.0 International License, which permits use, sharing, adaptation, distribution and reproduction in any medium or format, as long as you give appropriate credit to the original author(s) and the source, provide a link to the Creative Commons licence, and indicate if changes were made. The images or other third party material in this article are included in the article's Creative Commons licence, unless indicated otherwise in a credit line to the material. If material is not included in the article's Creative Commons licence and your intended use is not permitted by statutory regulation or exceeds the permitted use, you will need to obtain permission directly from the copyright holder. To view a copy of this licence, visit http://creativecommons.org/licenses/by/4.0/.
About this article
Cite this article
Jové-Llopis, E., Trujillo-Baute, E. Escaping the Energy Poverty Trap: Policy Assessment. Environ Resource Econ (2024). https://doi.org/10.1007/s10640-024-00918-2
Accepted:
Published:
DOI: https://doi.org/10.1007/s10640-024-00918-2