Abstract
In this paper, we propose an effective Bayesian subset selection method for the double-threshold-variable autoregressive moving-average (DT-ARMA) models. The usual complexity of estimation is increased mainly by capturing the correlation between two threshold variables and including moving-average terms in the model. By adopting the stochastic search variable selection method, combined with the Gibbs sampler and Metropolis-Hastings algorithm, we can simultaneously estimate the unknown parameters and select the best subset model from a large number of possible models. The simulation experiments illustrate that the proposed approach performs well. In applications, two real data sets are analyzed by the proposed method, and the fitted DT-ARMA model is better than the double-threshold autoregressive (DT-AR) model from the view of parsimony.















Similar content being viewed by others
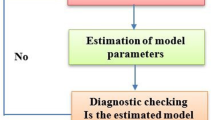
References
Amendola, A., Niglio, M., & Vitale, C. (2006). The moments of SETARMA models. Statistics and Probability Letters, 76, 625–633.
Bauwens, L., & Lubrano, M. (1998). Bayesian inference on GARCH models using Gibbs sampler. The Econometrics Journal, 1, 23–46.
Brockwell, P., Liu, J., & Tweedie, R. L. (1992). On the existence of stationary threshold autoregressive moving-average processes. Journal of Time Series Analysis, 13, 95–107.
Brooks, C., & Garrett, I. (2002). Can we explain the dynamics of the UK FTSE 100 stock and stock index futures markets. Applied Financial Economics, 12, 25–31.
Chen, C. W. S., & Lee, J. C. (1995). Bayesian inference of threshold autoregressive models. Journal of Time Series Analysis, 16, 483–492.
Chen, C. W. S., Liu, F. C., & Gerlach, R. (2011). Bayesian subset selection for threshold autoregressive moving-average models. Computational Statistics, 26, 1–30.
Chen, H., Chong, T. T. L., & Bai, J. (2012). Theory and applications of the model with two threshold variables. Econometric Reviews, 31(2), 142–170.
De Gooijer, G. (1998). On the threshold moving-average models. Journal of Time Series Analysis, 19, 1–18.
Durlauf, S. N., & Johnson, P. A. (1995). Multiple regimes and cross-country growth behavior. Journal of Applied Econometrics, 10, 365–384.
Geman, S., & Geman, D. (1984). Stochastic relaxation, Gibbs distribution and the Bayesian restoration of images. IEEE Transactions on Pattern Analysis and Machine Intelligence, 6, 721–741.
George, E. I., & McCulloch, R. E. (1993). Variable selection via Gibbs sampling. Journal of the American Statistical Association, 88, 881–889.
Geweke, J. (1989). Exact predictive densities for linear models with ARCH disturbances. Journal of Econometrics, 40, 63–86.
Goracci, G. (2020). An empirical study on the parsimony and descriptive power of TARMA models. Statistical Methods and Applications. https://doi.org/10.1007/s10260-020-00516-8.
Hastings, W. K. (1970). Monte-Carlo sampling methods using Markov chains and their applications. Biometrika, 57, 97–109.
Ismail, M. A., & Charif, H. A. (2003). Bayesian inference for threshold moving average models. METRON, 1, 119–132.
Ling, S. (1999). On the probabilistic properties of a double threshold ARMA conditional heteroskedastic model. Journal of Applied probability, 36, 688–705.
Ling, S., & Tong, H. (2005). Testing a linear moving-average model against threshold moving-average models. The Annals of Statistics, 33, 2529–2552.
Ling, S., Tong, H., & Li, D. (2007). Ergodicity and invertibility of threshold moving-average models. Bernoulli, 13, 161–168.
Mcculloch, R. E. and Tsay, R. S. (1993). Bayesian analysis of threshold autoregressive processes with a random number of regimes. In Computing Science and Statistics. Proceedings of the 25th Symposium on the Interface, Fairfax Station, VA: Interface Foundation of North America, pp. 253–262.
Metropolis, N., Rosenbluth, A. W., Rosenbluth, M. N., & Teller, E. (1953). Equations of state calculations by fast computing machines. Journal of Chemical Physics, 21, 1087–1091.
Ni, S., Xia, Q., & Liu, J. (2018). Bayesian subset selection for two-threshold variable autoregressive models. Studies in Nonlinear Dynamics and Econometrics, 22, 1–16.
Pan, J., Xia, Q., & Liu, J. (2017). Bayesian analysis of multiple thresholds autoregressive model. Computational Statistics, 32, 1–19.
Ritter, C., & Tanner, M. A. (1992). Facilitating the Gibbs sampler: The Gibbs stopper and the Griddy–Gibbs sampler. Journal of the American Statistical Association, 87, 861–868.
Sáfadi, T., & Morettin, P. A. (2000). Bayesian analysis of threshold autoregressive moving average models. Sankyã, 62, 353–371.
So, M. K. P., & Chen, C. W. S. (2003). Subset threshold autoregression. Journal of Forecasting, 22, 49–66.
Tiao, G. C., & Tsay, R. S. (1994). Some advances in nonlinear and adaptive modeling in time-series. Journal of Forecasting, 13, 109–131.
Tong, H. (1978). On a threshold model. In C. H. Chen (Ed.), Pattern recognition and signal processing. Sijthoff & Noordhoff
Tong, H. (1990). Non-linear time series: A dynamical system approach. Oxford University Press.
Tong, H., & Lim, K. S. (1980). Threshold autoregression, limit cycles and cyclical data (with discussion). Journal of the Royal Statistical Society, Series B, 42, 245–292.
Tsay, R. S. (1989). Testing and modeling threshold autoregressive process. Journal of the American Statistical Association, 84, 231–240.
Tsay, R. S. (2005). Analysis of financial time series. 2nd Edn. Wiley.
Xia, Q., Pan, J., Zhang, Z., & Liu, J. (2010). A Bayesian nonlinearity test for threshold moving average models. Journal of Time Series Analysis, 31, 329–336.
Xia, Q., Wong, H., Liu, J., & Liang, R. (2017). Bayesian analysis of power-transformed and threshold GARCH models: A Griddy–Gibbs sampler approach. Computational Economics, 50, 353–372.
Zhang, X., Li, D. and Tong, H. (2020). On the least squares estimation of 2-threshold-variable autoregressive models. Working paper.
Funding
The research of Qiang Xia was supported by the Major Research Plan of the National Natural Science Foundation of China (No.91746102) and Ministry of Education in China Project of Humanities and Social Sciences (No.17YJA910002). The research of Kun Liang was supported by Ministry of Education in China Project of Humanities and Social Sciences (No.18YJC630082) and the National Science Foundation of Anhui Province of China (1908085QG307). The research of Dabing Zhang was supported by the National Natural Science Foundation of China (No.71971089).
Author information
Authors and Affiliations
Corresponding author
Ethics declarations
Conflict of interest
We wish to confirm that there are no known conflict of interest associated with this publication. No conflict of interest exits in the submission of this manuscript, and manuscript is approved by all authors for publication.
Informed Consent
I would like to declare on behalf of my co-authors that the work described was original research that has not been published previously, and not under consideration for publication elsewhere, in whole or in part. All the authors listed have approved the
Additional information
Publisher's Note
Springer Nature remains neutral with regard to jurisdictional claims in published maps and institutional affiliations.
Rights and permissions
About this article
Cite this article
Zheng, X., Liang, K., Xia, Q. et al. Best Subset Selection for Double-Threshold-Variable Autoregressive Moving-Average Models: The Bayesian Approach. Comput Econ 59, 1175–1201 (2022). https://doi.org/10.1007/s10614-021-10124-7
Accepted:
Published:
Issue Date:
DOI: https://doi.org/10.1007/s10614-021-10124-7