Abstract
We perform classification, ranking and mapping of body sway parameters from static posturography data of patients using recent machine-learning and data-mining techniques. Body sway is measured in 293 individuals with the clinical diagnoses of acute unilateral vestibulopathy (AVS, n = 49), distal sensory polyneuropathy (PNP, n = 12), anterior lobe cerebellar atrophy (CA, n = 48), downbeat nystagmus syndrome (DN, n = 16), primary orthostatic tremor (OT, n = 25), Parkinson’s disease (PD, n = 27), phobic postural vertigo (PPV n = 59) and healthy controls (HC, n = 57). We classify disorders and rank sway features using supervised machine learning. We compute a continuous, human-interpretable 2D map of stance disorders using t-stochastic neighborhood embedding (t-SNE). Classification of eight diagnoses yielded 82.7% accuracy [95% CI (80.9%, 84.5%)]. Five (CA, PPV, AVS, HC, OT) were classified with a mean sensitivity and specificity of 88.4% and 97.1%, while three (PD, PNP, and DN) achieved a mean sensitivity of 53.7%. The most discriminative stance condition was ranked as “standing on foam-rubber, eyes closed”. Mapping of sway path features into 2D space revealed clear clusters among CA, PPV, AVS, HC and OT subjects. We confirm previous claims that machine learning can aid in classification of clinical sway patterns measured with static posturography. Given a standardized, long-term acquisition of quantitative patient databases, modern machine learning and data analysis techniques help in visualizing, understanding and utilizing high-dimensional sensor data from clinical routine.




Similar content being viewed by others
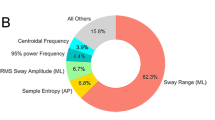
Abbreviations
- ANN:
-
Artificial neural network
- AVS:
-
Acute unilateral vestibulopathy
- CA:
-
Anterior lobe cerebella atrophy
- CI:
-
Confidence interval
- DN:
-
Downbeat nystagmus syndrome
- kNN:
-
k-Nearest-neighbors
- MDI:
-
Mean decrease in impurity
- HC:
-
Healthy controls
- OT:
-
Primary orthostatic tremor
- PCA:
-
Principal component analysis
- PD:
-
Parkinson’s disease
- PNP:
-
Sensory polyneuropathy
- RMS:
-
Root-mean-square
- SC:
-
Stacking classifier
- SVM:
-
Support vector machine
- t-SNE:
-
t-Stochastic neighborhood embedding
References
Dieterich M, Brandt T (2015) The bilateral central vestibular system: Its pathways, functions, and disorders. Ann N Y Acad Sci. 1343:10–26
Brandt T, Dieterich M (2017) The dizzy patient: don’t forget disorders of the central vestibular system. Nat Rev Neurol. 13:352–362
Staab JP, Eckhardt-Henn A, Horii A, Jacob R, Strupp M, Brandt T et al (2017) Diagnostic criteria for persistent postural-perceptual dizziness (PPPD): consensus document of the committee for the classification of vestibular disorders of the Bárány Society. J Vestib Res. 27:191–208
Black FO (2001) What can posturography tell us about vestibular function? Ann N Y Acad Sci. 942:446–464
de Waele C. Vestibular tests: static and dynamic posturography BT—encyclopedia of neuroscience. In: Binder MD, Hirokawa N, Windhorst U (eds) Springer, Berlin. 2009;4217–8.
Monsell EM, Furman JM, Herdman SJ, Konrad HR, Shepard NT (1997) Computerized dynamic platform posturography. Otolaryngol Head Neck Surg 117:394–398
Krafczyk S, Tietze S, Swoboda W, Valkovi P, Brandt T (2006) Artificial neural network: a new diagnostic posturographic tool for disorders of stance. Clin Neurophysiol. 117:1692–1698
Fioretti S, Scocco M, Ladislao L, Ghetti G, Rabini RA (2010) Identification of peripheral neuropathy in type-2 diabetic subjects by static posturography and linear discriminant analysis. Gait Posture. 32:317–320
Howcroft J, Lemaire ED, Kofman J, McIlroy WE (2017) Elderly fall risk prediction using static posturography. PLoS One 12:1–13
Saripalle SK, Paiva GC, Derakhshani RR, King GW, Lovelace CT (2014) Classification of body movements based on posturographic data. Hum Mov Sci. 33:238–250
Vonk J, Horlings CGC, Allum JHJ (2010) Differentiating malingering balance disorder patients from healthy controls, compensated unilateral vestibular loss, and whiplash patients using stance and gait posturography. Audiol Neurotol. 15:261–272
Redfern MS, Furman JM (1994) Postural sway of patients with vestibular disorders during optic flow. J Vestib Res. 4:221–230
Diener HC, Dichgans J, Bacher M, Gompf B (1984) Quantification of postural sway in normals and patients with cerebellar diseases. Clin Neurophysiol. Elsevier 57:134–142
Helmchen C, Kirchhoff J-B, Göttlich M, Sprenger A (2017) Postural ataxia in cerebellar downbeat nystagmus: its relation to visual, proprioceptive and vestibular signals and cerebellar atrophy. PLoS One 12:e0168808
Rigby HB, Rigby MH, Caviness JN (2015) Orthostatic tremor: a spectrum of fast and slow frequencies or distinct entities? Tremor Other Hyperkinet Mov (N Y). 5:324
Schöberl F, Feil K, Xiong G, Bartenstein P, la Fougére C, Jahn K et al (2017) Pathological ponto-cerebello-thalamo-cortical activations in primary orthostatic tremor during lying and stance. Brain 140:83–97
Ickenstein GW, Ambach H, Klöditz A, Koch H, Isenmann S, Reichmann H et al (2012) Static posturography in aging and Parkinson’s disease. Front Aging Neurosci. 4:20
Brandt T, Strupp M, Novozhilov S, Krafczyk S. Artificial neural network posturography detects the transition of vestibular neuritis to phobic postural vertigo. J. Neurol. 2012. p. 182–4.
Allum JHJ, Bloem BR, Carpenter MG, Honegger F (2001) Differential diagnosis of proprioceptive and vestibular deficits using dynamic support-surface posturography. Gait Posture. 14:217–226
Schwesig R, Fischer D, Becker S, Lauenroth A (2014) Intraobserver reliability of posturography in patients with vestibular neuritis. Somatosens Mot Res. 31:28–34
Bronstein AM, Guerraz M (1999) Visual-vestibular control of posture and gait: physiological mechanisms and disorders. Curr Opin Neurol. 12:5–11
Deuschl G, Bain P, Brin M (1998) Consensus statement of the movement disorder society on tremor. Ad Hoc Scientific Committee. Mov Disord. 13:2–23
Strupp M, Hufner K, Sandmann R, Zwergal A, Dieterich M, Jahn K et al (2011) Central oculomotor disturbances and nystagmus: a window into the brainstem and cerebellum. Dtsch Arztebl Int. 108:197–204
Strupp M, Brandt T (1999) Vestibular neuritis. Adv Otorhinolaryngol. 55:111–136
Gelb DJ, Oliver E, Gilman S (1999) Diagnostic criteria for Parkinson disease. Arch Neurol. 56:33–39
Richardson JK, Thies S, Ashton-Miller JA (2008) An exploration of step time variability on smooth and irregular surfaces in older persons with neuropathy. Clin Biomech. 23:349–356
Brandt T (1996) Phobic postural vertigo. Neurology. 46:1515–1519
Hufschmidt A, Dichgans J, Mauritz K-H, Hufschmidt M (1980) Some methods and parameters of body sway quantification and their neurological applications. Arch Psychiatr Nervenkr. 228:135–150
Brandt T, Krafczyk S, Malsbenden I (1981) Postural imbalance with head extension: improvement by training as a model for ataxia therapy. Ann N Y Acad Sci. 374:636–649
Caruana R, Karampatziakis N, Yessenalina A. An empirical evaluation of supervised learning in high dimensions. Proc 25th Int Conf Mach Learn-ICML ’08. 2008. p. 96–103.
Yu H-F, Huang F-L, Lin C-J (2011) Dual coordinate descent methods for logistic regression and maximum entropy models. Mach Learn. 85:41–75
Altman NS (1992) An Introduction to Kernel and Nearest-Neighbor Nonparametric Regression. Am Stat. 46:175–185
Hinton GE (1989) Connectionist learning procedures. Artif Intell. 40:185–234
Smola AJ, Sch B, Schölkopf B (2004) A tutorial on support vector regression. Stat Comput. 14:199–222
Criminisi, A, Konukoglu E, Shotton J (2011) Decision forests for classification, regression, density estimation manifold learning and semi-supervised learning microsoft technical report
Geurts P, Ernst D, Wehenkel L (2006) Extremely randomized trees. Mach Learn. 63:3–42
Pedregosa F, Varoquaux G, Gramfort A, Michel V, Thirion B, Grisel O et al (2011) Scikit-learn: machine learning in python. J Mach Learn Res. 12:2825–2830
Wolpert DH (1992) Stacked generalization. Neural Netw. 5:241–259
Breiman L, Friedman JH, Olshen RA, Stone CJ. Classification and Regression Trees. ed. 1. Wadsworth Stat. Ser. Chapman and Hall/CRC; 1984.
van der Maaten L, Hinton GE (2008) Visualizing high-dimensional data using t-SNE. J Mach Learn Res. 9:2579–2605
Jones E, Oliphant T, Peterson P, others. SciPy: Open source scientific tools for Python [Internet]. 2001. https://www.scipy.org/
Gallea C, Popa T, García-Lorenzo D, Valabregue R, Legrand AP, Apartis E et al (2016) Orthostatic tremor: a cerebellar pathology? Brain 139:2182–2197
Hageman PA, Leibowitz JM, Blanke D (1995) Age and gender effects on postural control measures. Arch Phys Med Rehabil. 76:961–965
Gill J, Allum JHJ, Carpenter MG, Held-Ziolkowska M, Adkin AL, Honegger F et al (2001) Trunk sway measures of postural stability during clinical balance tests: effects of age. J Gerontol Ser A Biol Sci Med Sci. 56:438–447
Feil K, Böttcher N, Guri F, Krafczyk S, Schöberl F, Zwergal A et al (2015) Long-term course of orthostatic tremor in serial posturographic measurement. Park Relat Disord. 21:905–910
Fung VSC, Sauner D, Day BL (2001) A dissociation between subjective and objective unsteadiness in primary orthostatic tremor. Brain 124:322–330
Wuehr M, Schlick C, Möhwald K, Schniepp R (2018) Walking in orthostatic tremor modulates tremor features and is characterized by impaired gait stability. Sci Rep. 8:14152
Kumar N, Kohlbecher S, Schneider E. A novel approach to video-based pupil tracking. Conf Proc—IEEE Int Conf Syst Man Cybern. 2009. pp 1255–62.
Kirsch V, Keeser D, Hergenroeder T, Erat O, Ertl-Wagner B, Brandt T et al (2016) Structural and functional connectivity mapping of the vestibular circuitry from human brainstem to cortex. Brain Struct Funct. 221:1291–1308
Pradhan C, Wuehr M, Akrami F, Neuhaeusser M, Huth S, Brandt T et al (2015) Automated classification of neurological disorders of gait using spatio-temporal gait parameters. J Electromyogr Kinesiol. 25:413–422
Acknowledgements
The study was supported by the German Federal Ministry of Education and Research (BMBF) in connection with the foundation of the German Center for Vertigo and Balance Disorders (DSGZ) (Grant number 01 EO 0901).
Author information
Authors and Affiliations
Corresponding author
Ethics declarations
Conflicts of interest
None of the authors have potential conflicts of interest to be disclosed.
Additional information
This manuscript is part of a supplement sponsored by the German Federal Ministry of Education and Research within the funding initiative for integrated research and treatment centers.
Rights and permissions
About this article
Cite this article
Ahmadi, SA., Vivar, G., Frei, J. et al. Towards computerized diagnosis of neurological stance disorders: data mining and machine learning of posturography and sway. J Neurol 266 (Suppl 1), 108–117 (2019). https://doi.org/10.1007/s00415-019-09458-y
Received:
Revised:
Accepted:
Published:
Issue Date:
DOI: https://doi.org/10.1007/s00415-019-09458-y