Abstract
This manuscript deals with the problem of passification of fractional-order neural networks (FONNs) with proportional delays and impulses in finite time. The primary contribution of this work lies in the fact that the concept of finite-time passivity (FTP) is extended for FONNs with and without impulses for the first time. At the fore set, the notion of FTP in terms of Lyapunov function is extended to fractional-order systems. Based on the proposed definition of FTP, sufficient conditions in terms of LMI are derived to ensure the FTP of the considered FONNs. Further to this, a new lemma using comparison principle is derived using which the definition of FTP for FONNs with impulses is put forth. From these results, some new set of LMI conditions for the considered system to be finite-time stable (FTS) is derived. Finally, theoretical results are verified via a numerical example.
Access this chapter
Tax calculation will be finalised at checkout
Purchases are for personal use only
Similar content being viewed by others
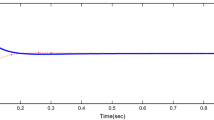
References
Kilbas, A., Srivastava, H., Trujillo, J.: Theory and Applications of Fractional Differential Equations. Elsevier, New York (2006)
Jahanbakhti, H.: A novel fractional-order neural network for model reduction of large-scale systems with fractional-order nonlinear structure. Soft Comput. 24, 13489–13499 (2020)
Wang, H., Yu, Y., Wen, G.: Stability analysis of fractional order Hopfield neural networks with time delays. Neural Netw. 55, 98–109 (2014)
Wan, L., Liu, Z.: Multiple \(O(t^{-\alpha })\) stability for fractional-order neural networks with time-varying delays. J. Franklin Inst. 357(17), 12742–12766 (2020)
Celik, V.: Bifurcation analysis of fractional order single cell with delay. Int. J. Bifurcat. Chaos 25(02), 1550020 (2015)
Zhou, L.: On the global dissipativity of a class of cellular neural networks with multi-pantograph delays. Adv. Artif. Neural Syst. 2011, 941426 (2011)
Zhou, L.: Delay-dependent and delay-independent passivity of a class of recurrent neural networks with impulse and multi-proportional delays. Neurocomputing 308, 235–244 (2018)
Zhou, L.: Dissipativity of a class of cellular neural networks with proportional delays. Nonlinear Dyn. 73, 1895–1903 (2013)
Zhou, L.: Delay-dependent exponential stability of cellular neural networks with multi-proportional delays. Neural Process. Lett. 38, 321–346 (2013)
Xiong, X., Tang, R., Yang, X.: Finite-time synchronization of memristive neural networks with proportional delay. Neural Process. Lett. 50, 1139–1152 (2019)
Hu, J., Sui, G.: Fixed-time control of static impulsive neural networks with infinite distributed delay and uncertainty. Commun. Nonlinear Sci. Numer. Simul. 78, 104848 (2019)
Li, H., Li, C., Huang, T., Zhang, W.: Fixed-time stabilization of impulsive Cohen-Grossberg BAM neural networks. Neural Netw. 98, 203–211 (2018)
Wu, A., Zeng, Z.: Passivity analysis of memristive neural networks with different memductance functions. Commun. Nonlinear Sci. Numer. Simul. 19, 274–285 (2014)
Wang, S., Cao, Y., Huang, T., Wen, S.: Passivity and passification of memristive neural networks with leakage term and time-varying delays. Appl. Math. Comput. 361, 294–310 (2019)
Velmurugan, G., Rakkiyappan, R., Lakshmanan, S.: Passivity analysis of memristor based complex valued neural networks with time-varying delays. Neural Process. Lett. 42, 517–540 (2015)
Jiang, B., Hu, Q., Friswell, M.I.: Fixed-time attitude control for rigid spacecraft with actuator saturation and faults. IEEE Trans. Control Syst. Technol. 24, 1892–1898 (2016)
Polyakov, A., Efimov, D., Perruquetti, W.: Robust stabilization of MIMO systems in finite/fixed-time. Int. J. Robust Nonlinear Control 26, 69–90 (2016)
Peng, X., Wu, H., Cao, J.: Global nonfragile synchronization in finite time for fractional order discontinuous neural networks with nonlinear growth activations. IEEE Trans. Neural Netw. Learn. Syst. 30(7), 2123–2137 (2018)
Wang, J., Wu, H., Huang, T.: Passivity based synchronization of a class of complex dynamic networks with time-varying delays. Automatica 56(1), 105–112 (2010)
Hou, M., Tan, F., Duan, G.: Finite-time passivity of dynamic systems. J. Franklin Inst. 353(18), 4870–4884 (2016)
Xiao, J., Zeng, Z.: Finite-time passivity of neural networks with time-varying delay. J. Franklin Inst. 357(4), 2437–2456 (2020)
Wang, Z., Cao, J., Lu, G., Abdel-Aty, M.: Fixed-time passification analysis of interconnected memristive reaction-diffusion neural networks. IEEE Trans. Netw. Sci. Eng. 7(3), 1814–1824 (2020)
Li, H.-L., Cao, J., Jiang, H., Alsaedi, A.: Graph theory based finite-time synchronization of fractional-order complex dynamical networks. J. Franklin Inst. 355(13), 5771–5789 (2018)
Li, C., Deng, W.: Remarks on fractional derivatives. Appl. Math. Comput. 187, 777–784 (2007)
Duarte-Mermoud, M.A., Aguila-Camacho, N., Gallegos, J.A., Castro-Linares, R.: Using general quadratic Lyapunov functions to prove Lyapunov uniform stability for fractional order systems. Commun. Nonlinear Sci. Numer. Simul. 22, 650–659 (2015)
Wang, L., Shen, Y., Ding, Z.: Finite-time stabilization of delayed neural networks. Neural Netw. 70, 74–80 (2015)
Wu, R., Fečkan, M.: Stabilty analysis of impulsive fractional-order systems by vector comparison principle. Nonlinear Dyn. 82, 2007–2019 (2015)
Wang, Z., Huang, X., Zhou, J.: A numerical method for delayed fractional-order differential equations: based on G-L definition. Appl. Math. Inf. Sci. 7(21), 525–529 (2013)
Pu, Y.-F., Yi, Z., Zhou, J.-L.: Fractional Hopfield neural networks: fractional dynamic associative recurrent neural networks. IEEE Trans. Neural Netw. Learn. Syst. 28(10), 2319–2333 (2017)
Acknowledgements
Funding: This work was supported by UGC-SAP (DSA-I), New Delhi, India, File No.: F.510/7/DSA-1/2015(SAP-I).
Author information
Authors and Affiliations
Editor information
Editors and Affiliations
Rights and permissions
Copyright information
© 2021 The Author(s), under exclusive license to Springer Nature Singapore Pte Ltd.
About this paper
Cite this paper
Padmaja, N., Balasubramaniam, P. (2021). Finite-Time Passification of Fractional-Order Recurrent Neural Networks with Proportional Delay and Impulses: an LMI Approach. In: Balasubramaniam, P., Ratnavelu, K., Rajchakit, G., Nagamani, G. (eds) Mathematical Modelling and Computational Intelligence Techniques. ICMMCIT 2021. Springer Proceedings in Mathematics & Statistics, vol 376. Springer, Singapore. https://doi.org/10.1007/978-981-16-6018-4_13
Download citation
DOI: https://doi.org/10.1007/978-981-16-6018-4_13
Published:
Publisher Name: Springer, Singapore
Print ISBN: 978-981-16-6017-7
Online ISBN: 978-981-16-6018-4
eBook Packages: Mathematics and StatisticsMathematics and Statistics (R0)