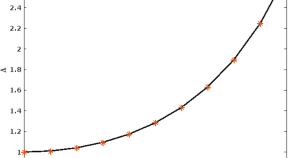
Collection
Emerging Trends in Artificial Intelligence-Based Mathematical Modeling for Model Predictive Control of Engineering Systems
- Submission status
- Closed
The fast pace of recent developments in the field of artificial intelligence (AI) has made it possible to apply different AI-based mathematical modeling techniques to various applications. One such application is model predictive control (MPC) of engineering systems, which is necessary to improve their operation and performance. The engineering of complex systems is a process of removing uncertainty from the system, with the objective of developing an optimal control algorithm. This increased complexity has driven us to reconsider our models and how we inform them. Since its inception, artificial intelligence (AI) has had a transformative impact on many disciplines in science, engineering, and medicine. The ability of AI-based mathematical modeling to create more accurate models based on appropriate features reduces the irreducibility of complex processes for mathematical optimization methods such as model predictive control (MPC). Mathematical modeling is important in developing efficient and effective control strategies for nonlinear systems. However, the state-of-the-art approaches lack the flexibility to accommodate uncertainties and dynamic system behaviors. Artificial intelligence (AI) has been used to address these challenges, but most classical AI techniques are limited by their computationally prohibitive nature.
This is a need for more research in Artificial Intelligence-Based Mathematical Modeling for Model Predictive Control of Engineering Systems. In the last two decades, artificial intelligence has been used in all major branches of science, including engineering and computer science. Improved decision-making in uncertainty has been an important issue in engineering over the past few decades, particularly due to its potential for improving system stability and tracking performance. The need for more research in artificial intelligence-based mathematical modeling for model predictive control of engineering systems is evident from the lack of developments in this area. It was found that conventional analytical models do not work well in the dynamic and stochastic environments of practical applications; accordingly, research has been conducted on alternative approaches to model predictive control (MPC). Unfortunately, the majority of research efforts have focused on applying tools of statistical analysis to existing plant models or to data collected by sensors. More attention should be given to developing new methodologies based on neural network and fuzzy systems technology, which offer improved mathematical modeling accuracy over conventional methods.
This special issue aims to provide a comprehensive overview of the latest advances in using artificial intelligence (AI) techniques for mathematical modeling and model predictive control (MPC) of engineering systems. The focus will be on emerging trends and innovative approaches that leverage AI techniques to improve the accuracy, robustness, and efficiency of mathematical models and MPC algorithms.
Editors
-
Dr. Shadi Mahmoud Faleh AlZu’bi
Dr. Shadi Mahmoud Faleh AlZu’bi received his Ph.D. (Electronic and Computer / Computer Science) in 2011 at Brunel University, West London, United Kingdom, M.Sc. (Telecommunication Networks Management) in 2008 from University of Technology, Sydney, Australia, and B.Sc. (Computer and Network Engineering) in 2007 from Jordan University of Science and Technology, Jordan. He is currently working as Associate Professor at Department of Computer Science, Faculty of Science and Information Technology, Al-Zaytoonah University of Jordan, Amman, Jordan.
-
Dr. Maysam Abbod
Dr. Maysam Abbod (MIET, CEng, SMIEEE, SFHEA) He received BSc degree in Electrical Engineering from University of Technology in 1987and PhD in Control Engineering from University of Sheffield in 1992. From 1993 to 2006 he was with the Department of Automatic Control and Systems Engineering at the University of Sheffield as a research associate and senior research fellow.
-
Dr. Ashraf Darwish
Dr. Ashraf Darwish is an Associate Professor of Computer Science and Acting as the Head of Mathematics and Computer Science Department at Faculty of Science, Helwan University, Egypt. He received the PhD degree in computer science from Saint Petersburg State University, Russian Federation in 2006. He received B.Sc. and M.Sc. in Mathematics from Faculty of Science, Tanta University, Egypt. He keeps in touch with his mathematical background through his research.
Articles (5 in this collection)
-
-
Adaptive backstepping control for sensor-less haptic joysticks with uncertainty parameters
Authors
- Tao Ni
- Panhong Zhang
- Zeren Zhao
- Content type: OriginalPaper
- Published: 11 March 2024
- Article: 6
-
Effective methods for numerical analysis of the simplest chaotic circuit model with Atangana–Baleanu Caputo fractional derivative
Authors (first, second and last of 6)
- Abdulrahman B. M. Alzahrani
- Rania Saadeh
- Ahmad Qazza
- Content type: OriginalPaper
- Published: 03 January 2024
- Article: 9
-
Two-phase analysis on radiative solar pump applications using MHD Eyring–Powell hybrid nanofluid flow with the non-Fourier heat flux model
Authors (first, second and last of 4)
- Seethi Reddy Reddisekhar Reddy
- Shaik Jakeer
- Kuppala R. Sekhar
- Content type: OriginalPaper
- Published: 21 December 2023
- Article: 7
-
A robust scheme for reduction of higher fractional-order systems
Authors (first, second and last of 4)
- Iqbal M. Batiha
- Nadia Allouch
- Shaher Momani
- Content type: OriginalPaper
- Published: 08 December 2023
- Article: 4